Iceberg基于Spark MergeInto语法实现数据的增量写入
SPARK SQL 基本语法
示例SQL如下
MERGE INTO target_table t
USING source_table s
ON s.id = t.id //这里是JOIN的关联条件
WHEN MATCHED AND s.opType = 'delete' THEN DELETE // WHEN条件是对当前行进行打标的匹配条件
WHEN MATCHED AND s.opType = 'update' THEN UPDATE SET id = s.id, name = s.name
WHEN NOT MATCHED AND s.opType = 'insert' THEN INSERT (key, value) VALUES (key, value)
Source表和target表,先按di列进行JOIN,然后对于关联后的结果集的每一行进行条件判断,如果opType=‘delete’,那么就删除当前行;如果是不匹配而且opType=‘insert’那么,就将source表中的数据插入到目标表。
Spark3.3 中定义的三种数据行的状态
package org.apache.spark.sql.catalyst.util
object RowDeltaUtils {// 新旧数据记录,Merge阶段,会为每一个结果行添加一个新的列,其列名就这个常量final val OPERATION_COLUMN: String = "__row_operation"final val DELETE_OPERATION: Int = 1final val UPDATE_OPERATION: Int = 2final val INSERT_OPERATION: Int = 3
}
源码跟踪
文章引用的代码来自Iceberg 1.0.x 和Spark 3.3版本。
一、重写逻辑计划树
运行时,如果发现当前SQL是MergeIntoIcebergTable,则会生成在生成优化的逻辑计划树时,应用如下的Rule,重写当前的Merge into 逻辑树:
object RewriteMergeIntoTable extends RewriteRowLevelIcebergCommand {private final val ROW_FROM_SOURCE = "__row_from_source"private final val ROW_FROM_TARGET = "__row_from_target"private final val ROW_ID = "__row_id"private final val ROW_FROM_SOURCE_REF = FieldReference(ROW_FROM_SOURCE)private final val ROW_FROM_TARGET_REF = FieldReference(ROW_FROM_TARGET)private final val ROW_ID_REF = FieldReference(ROW_ID)override def apply(plan: LogicalPlan): LogicalPlan = plan resolveOperators {// ... 跳过其它情况下的匹配case m @ MergeIntoIcebergTable(aliasedTable, source, cond, matchedActions, notMatchedActions, None)if m.resolved && m.aligned =>EliminateSubqueryAliases(aliasedTable) match {case r @ DataSourceV2Relation(tbl: SupportsRowLevelOperations, _, _, _, _) =>val table = buildOperationTable(tbl, MERGE, CaseInsensitiveStringMap.empty())val rewritePlan = table.operation match {case _: SupportsDelta =>// 构建增量逻辑计划,table指的是Iceberg目标表,source指的是新数据buildWriteDeltaPlan(r, table, source, cond, matchedActions, notMatchedActions)case _ =>// 否则就是COW模式buildReplaceDataPlan(r, table, source, cond, matchedActions, notMatchedActions)}m.copy(rewritePlan = Some(rewritePlan))case p =>throw new AnalysisException(s"$p is not an Iceberg table")}
}// build a rewrite plan for sources that support row deltas
private def buildWriteDeltaPlan(relation: DataSourceV2Relation,operationTable: RowLevelOperationTable,source: LogicalPlan,cond: Expression,matchedActions: Seq[MergeAction], // 通过ResolveMergeIntoTableReferences规则,从merge sql语句中解析出来,merge行为,例如UPDATE关键字对应于UpdateActionnotMatchedActions: Seq[MergeAction]): WriteDelta = {// resolve all needed attrs (e.g. row ID and any required metadata attrs)val rowAttrs = relation.outputval rowIdAttrs = resolveRowIdAttrs(relation, operationTable.operation)val metadataAttrs = resolveRequiredMetadataAttrs(relation, operationTable.operation)// construct a scan relation and include all required metadata columns// operaionTable表示的待写入的目标表,这是会根据关联条件,构建一个目标表的scan relationval readRelation = buildRelationWithAttrs(relation, operationTable, rowIdAttrs ++ metadataAttrs)val readAttrs = readRelation.output// project an extra column to check if a target row exists after the join// 为目标表的数据添加一列,表示该行数据来自于目标表val targetTableProjExprs = readAttrs :+ Alias(TrueLiteral, ROW_FROM_TARGET)()// 生成目标表的输出数据val targetTableProj = Project(targetTableProjExprs, readRelation)// project an extra column to check if a source row exists after the join// 为新的数据添加一列,表示该行数据来自于新的输入val sourceTableProjExprs = source.output :+ Alias(TrueLiteral, ROW_FROM_SOURCE)()val sourceTableProj = Project(sourceTableProjExprs, source)// use inner join if there is no NOT MATCHED action, unmatched source rows can be discarded// use right outer join in all other cases, unmatched source rows may be needed// also disable broadcasts for the target table to perform the cardinality checkval joinType = if (notMatchedActions.isEmpty) Inner else RightOuterval joinHint = JoinHint(leftHint = Some(HintInfo(Some(NO_BROADCAST_HASH))), rightHint = None)// 将从目标表读取的数据,与新的、待写入的来源表的数据进行JOIN,如果决定忽略不匹配的字段(丢弃)那么会使用inner join,// 否则使用right join,由于目标表在JOIN左边,因此也就意味着,最终的Join结果是以新数据为基准,如果成功与目标// 表的数据行关联,则说明是要update的数据行;没有新数据没有关联到目标表的数据,则说明是该行数据记录属于新增insert的数据行。val joinPlan = Join(NoStatsUnaryNode(targetTableProj), sourceTableProj, joinType, Some(cond), joinHint)val deleteRowValues = buildDeltaDeleteRowValues(rowAttrs, rowIdAttrs)val metadataReadAttrs = readAttrs.filterNot(relation.outputSet.contains)val matchedConditions = matchedActions.map(actionCondition)// 创建匹配的数据行的输出meta信息,MergeRowsExec会根据这些信息,生成数据行的投影器,并与matchedConditions合并生成一个matchedPairs的二元组,// val matchedPairs = matchedPreds zip matchedProjs// MergeRowsExec就以此二元组来应用到数据记录上,得到merge后的、带有操作类型的internal rowval matchedOutputs = matchedActions.map(deltaActionOutput(_, deleteRowValues, metadataReadAttrs))val notMatchedConditions = notMatchedActions.map(actionCondition)val notMatchedOutputs = notMatchedActions.map(deltaActionOutput(_, deleteRowValues, metadataReadAttrs))// 为merge后的数据添加新的一列,即operation_column,用于标记每一行的记录的类型// final val OPERATION_COLUMN: String = "__row_operation"// final val DELETE_OPERATION: Int = 1// final val UPDATE_OPERATION: Int = 2// final val INSERT_OPERATION: Int = 3val operationTypeAttr = AttributeReference(OPERATION_COLUMN, IntegerType, nullable = false)()val rowFromSourceAttr = resolveAttrRef(ROW_FROM_SOURCE_REF, joinPlan)val rowFromTargetAttr = resolveAttrRef(ROW_FROM_TARGET_REF, joinPlan)// merged rows must contain values for the operation type and all read attrsval mergeRowsOutput = buildMergeRowsOutput(matchedOutputs, notMatchedOutputs, operationTypeAttr +: readAttrs)// 生成一个MergeRows的逻辑计划节点,joinPlan作为其上游节点,会对应生成MergeRowsExec物理算子val mergeRows = MergeRows(isSourceRowPresent = IsNotNull(rowFromSourceAttr),isTargetRowPresent = if (notMatchedActions.isEmpty) TrueLiteral else IsNotNull(rowFromTargetAttr),matchedConditions = matchedConditions,matchedOutputs = matchedOutputs,notMatchedConditions = notMatchedConditions,notMatchedOutputs = notMatchedOutputs,// only needed if emitting unmatched target rowstargetOutput = Nil,rowIdAttrs = rowIdAttrs,performCardinalityCheck = isCardinalityCheckNeeded(matchedActions),emitNotMatchedTargetRows = false,output = mergeRowsOutput,joinPlan)// build a plan to write the row delta to the tableval writeRelation = relation.copy(table = operationTable)val projections = buildMergeDeltaProjections(mergeRows, rowAttrs, rowIdAttrs, metadataAttrs)// WriteDelta会对应生成WriteDeltaExec物理算子,写出增量数据到目标表WriteDelta(writeRelation, mergeRows, relation, projections)
}
二、Merge rows合并结果集
case class MergeRowsExec(…) {
// 在每一个Partition数据集上进行验证,根据匹配表达式的结果为每一行数据记录添加标记
private def processPartition(rowIterator: Iterator[InternalRow]): Iterator[InternalRow] = {val inputAttrs = child.outputval isSourceRowPresentPred = createPredicate(isSourceRowPresent, inputAttrs)val isTargetRowPresentPred = createPredicate(isTargetRowPresent, inputAttrs)val matchedPreds = matchedConditions.map(createPredicate(_, inputAttrs))val matchedProjs = matchedOutputs.map {case output if output.nonEmpty => Some(createProjection(output, inputAttrs))case _ => None}// matchedPreds,即一个或多个predicate用于判定当前行是不是满足 给定的条件// matchedProjs,即一个UnsafeProjection的对象,可以将一个InternalRow写出成一个UnsafeRow,并且带有具体的更新类型,UPDATE/INSERT/DELETEval matchedPairs = matchedPreds zip matchedProjsval notMatchedPreds = notMatchedConditions.map(createPredicate(_, inputAttrs))val notMatchedProjs = notMatchedOutputs.map {case output if output.nonEmpty => Some(createProjection(output, inputAttrs))case _ => None}val nonMatchedPairs = notMatchedPreds zip notMatchedProjsval projectTargetCols = createProjection(targetOutput, inputAttrs)val rowIdProj = createProjection(rowIdAttrs, inputAttrs)// This method is responsible for processing a input row to emit the resultant row with an// additional column that indicates whether the row is going to be included in the final// output of merge or not.// 1. Found a target row for which there is no corresponding source row (join condition not met)// - Only project the target columns if we need to output unchanged rows// 2. Found a source row for which there is no corresponding target row (join condition not met)// - Apply the not matched actions (i.e INSERT actions) if non match conditions are met.// 3. Found a source row for which there is a corresponding target row (join condition met)// - Apply the matched actions (i.e DELETE or UPDATE actions) if match conditions are met.// 处理每一行数据,注意这里的结果集是来自于target RIGHT OUTER JOIN source的结果,因此如果目标表的数据行没有出现,// 说明当前行是不匹配的;如果source表中的行不存在,则说明行是不匹配的;否则就是目标的行和source表中的行都出现了,// 说明当前行是匹配的。// 总之,对于最终的结果,source表的数据行有三种状态(新增数据),UPDATE/DELETE/INSERT。def processRow(inputRow: InternalRow): InternalRow = {// 如果忽略不匹配的行或是源数据行不匹配if (emitNotMatchedTargetRows && !isSourceRowPresentPred.eval(inputRow)) {projectTargetCols.apply(inputRow)} else if (!isTargetRowPresentPred.eval(inputRow)) {// 如果是不匹配的数据行,生成一个新的row,并带有相应的操作类型,一般是INSERT,作为第一个字段applyProjection(nonMatchedPairs, inputRow)} else {// 如果是匹配的数据行,则生成一个新的row,并带有相应的操作类型,一般是DELETE或是UPDATE,作为第一个字段applyProjection(matchedPairs, inputRow)}}var lastMatchedRowId: InternalRow = nulldef processRowWithCardinalityCheck(inputRow: InternalRow): InternalRow = {val isSourceRowPresent = isSourceRowPresentPred.eval(inputRow)val isTargetRowPresent = isTargetRowPresentPred.eval(inputRow)if (isSourceRowPresent && isTargetRowPresent) {val currentRowId = rowIdProj.apply(inputRow)if (currentRowId == lastMatchedRowId) {throw new SparkException("The ON search condition of the MERGE statement matched a single row from " +"the target table with multiple rows of the source table. This could result " +"in the target row being operated on more than once with an update or delete " +"operation and is not allowed.")}lastMatchedRowId = currentRowId.copy()} else {lastMatchedRowId = null}if (emitNotMatchedTargetRows && !isSourceRowPresent) {projectTargetCols.apply(inputRow)} else if (!isTargetRowPresent) {applyProjection(nonMatchedPairs, inputRow)} else {applyProjection(matchedPairs, inputRow)}}val processFunc: InternalRow => InternalRow = if (performCardinalityCheck) {processRowWithCardinalityCheck} else {processRow}rowIterator.map(processFunc).filter(row => row != null)
}}
三、增量数据写出
/*** Physical plan node to write a delta of rows to an existing table.*/
case class WriteDeltaExec(query: SparkPlan,refreshCache: () => Unit,projections: WriteDeltaProjections,write: DeltaWrite) extends ExtendedV2ExistingTableWriteExec[DeltaWriter[InternalRow]] {override lazy val references: AttributeSet = query.outputSetoverride lazy val stringArgs: Iterator[Any] = Iterator(query, write)// 创建增量写出数据的任务,详细定义见后面DeltaWithMetadataWritingSparkTaskoverride lazy val writingTask: WritingSparkTask[DeltaWriter[InternalRow]] = {DeltaWithMetadataWritingSparkTask(projections)}override protected def withNewChildInternal(newChild: SparkPlan): WriteDeltaExec = {copy(query = newChild)}
}case class DeltaWithMetadataWritingSparkTask(projs: WriteDeltaProjections) extends WritingSparkTask[DeltaWriter[InternalRow]] {private lazy val rowProjection = projs.rowProjection.orNullprivate lazy val rowIdProjection = projs.rowIdProjectionprivate lazy val metadataProjection = projs.metadataProjection.orNull// InternalRow来自于Merge后的结果,每一行的第一个字段,标记了当前行的操作类型override protected def writeFunc(writer: DeltaWriter[InternalRow], row: InternalRow): Unit = {val operation = row.getInt(0)operation match {case DELETE_OPERATION =>rowIdProjection.project(row)metadataProjection.project(row)// 如果当前数据行被 标记为DELETE,那么就执行删除操作,如果数据来自分区表,那么底层调用PartitionedDeltaWriter::delete(…)方法writer.delete(metadataProjection, rowIdProjection)case UPDATE_OPERATION =>rowProjection.project(row)rowIdProjection.project(row)metadataProjection.project(row)// 同上,如果数据来自分区表,那么底层调用PartitionedDeltaWriter::update(…)方法writer.update(metadataProjection, rowIdProjection, rowProjection)case INSERT_OPERATION =>rowProjection.project(row)writer.insert(rowProjection)case other =>throw new SparkException(s"Unexpected operation ID: $other")}}
}
/** Spark写出任务,公共接口,提供统一的写出过程 */
trait WritingSparkTask[W <: DataWriter[InternalRow]] extends Logging with Serializable {protected def writeFunc(writer: W, row: InternalRow): Unitdef run(writerFactory: DataWriterFactory,context: TaskContext,iter: Iterator[InternalRow],useCommitCoordinator: Boolean,customMetrics: Map[String, SQLMetric]): DataWritingSparkTaskResult = {val stageId = context.stageId()val stageAttempt = context.stageAttemptNumber()val partId = context.partitionId()val taskId = context.taskAttemptId()val attemptId = context.attemptNumber()val dataWriter = writerFactory.createWriter(partId, taskId).asInstanceOf[W]var count = 0L// write the data and commit this writer.Utils.tryWithSafeFinallyAndFailureCallbacks(block = {while (iter.hasNext) { // 遍历RDD中的每一行if (count % CustomMetrics.NUM_ROWS_PER_UPDATE == 0) {CustomMetrics.updateMetrics(ArraySeq.unsafeWrapArray(dataWriter.currentMetricsValues), customMetrics)}// Count is here.count += 1// 即调用DeltaWithMetadataWritingSparkTask::writeFunc(..)方法,执行真正的写出writeFunc(dataWriter, iter.next())}CustomMetrics.updateMetrics(ArraySeq.unsafeWrapArray(dataWriter.currentMetricsValues), customMetrics)// 数据写出完成,向Spark中的OutputCommitCoordinator提交val msg = if (useCommitCoordinator) {val coordinator = SparkEnv.get.outputCommitCoordinatorval commitAuthorized = coordinator.canCommit(stageId, stageAttempt, partId, attemptId)if (commitAuthorized) {logInfo(s"Commit authorized for partition $partId (task $taskId, attempt $attemptId, " +s"stage $stageId.$stageAttempt)")dataWriter.commit()} else {val commitDeniedException = QueryExecutionErrors.commitDeniedError(partId, taskId, attemptId, stageId, stageAttempt)logInfo(commitDeniedException.getMessage)// throwing CommitDeniedException will trigger the catch block for abortthrow commitDeniedException}} else {logInfo(s"Writer for partition ${context.partitionId()} is committing.")dataWriter.commit()}logInfo(s"Committed partition $partId (task $taskId, attempt $attemptId, " +s"stage $stageId.$stageAttempt)")DataWritingSparkTaskResult(count, msg)})(catchBlock = {// If there is an error, abort this writerlogError(s"Aborting commit for partition $partId (task $taskId, attempt $attemptId, " +s"stage $stageId.$stageAttempt)")dataWriter.abort()logError(s"Aborted commit for partition $partId (task $taskId, attempt $attemptId, " +s"stage $stageId.$stageAttempt)")}, finallyBlock = {dataWriter.close()})}
}
四、分区表数据的增量写出
private static class PartitionedDeltaWriter extends DeleteAndDataDeltaWriter {private final PartitionSpec dataSpec;private final PartitionKey dataPartitionKey;private final InternalRowWrapper internalRowDataWrapper;PartitionedDeltaWriter(Table table,SparkFileWriterFactory writerFactory,OutputFileFactory dataFileFactory,OutputFileFactory deleteFileFactory,Context context) {super(table, writerFactory, dataFileFactory, deleteFileFactory, context);this.dataSpec = table.spec();this.dataPartitionKey = new PartitionKey(dataSpec, context.dataSchema());this.internalRowDataWrapper = new InternalRowWrapper(context.dataSparkType());}// 删除旧的数据记录,这里是写出position delete file,此方法实际上是在父类当中的定义的,具体的注释,见DeleteAndDataDeltaWriter类的解析
@Override
public void delete(InternalRow meta, InternalRow id) throws IOException {int specId = meta.getInt(specIdOrdinal);PartitionSpec spec = specs.get(specId);InternalRow partition = meta.getStruct(partitionOrdinal, deletePartitionRowWrapper.size());StructProjection partitionProjection = deletePartitionProjections.get(specId);partitionProjection.wrap(deletePartitionRowWrapper.wrap(partition));String file = id.getString(fileOrdinal);long position = id.getLong(positionOrdinal);delegate.delete(file, position, spec, partitionProjection);
}@Overridepublic void update(InternalRow meta, InternalRow id, InternalRow row) throws IOException {delete(meta, id); // 删除旧的数据记录,这里是写出position delete filedataPartitionKey.partition(internalRowDataWrapper.wrap(row));// 写入新的数据行,delegate实际上是一个DeleteAndDataDeltaWriter的实例delegate.update(row, dataSpec, dataPartitionKey);}@Overridepublic void insert(InternalRow row) throws IOException {dataPartitionKey.partition(internalRowDataWrapper.wrap(row));delegate.insert(row, dataSpec, dataPartitionKey);}
}
DeleteAndDataDeltaWriter:删除和增量更新的抽象基类
private abstract static class DeleteAndDataDeltaWriter extends BaseDeltaWriter {protected final PositionDeltaWriter<InternalRow> delegate;private final FileIO io;private final Map<Integer, PartitionSpec> specs;private final InternalRowWrapper deletePartitionRowWrapper;private final Map<Integer, StructProjection> deletePartitionProjections;private final int specIdOrdinal;private final int partitionOrdinal;private final int fileOrdinal;private final int positionOrdinal;private boolean closed = false;DeleteAndDataDeltaWriter(Table table,SparkFileWriterFactory writerFactory,OutputFileFactory dataFileFactory,OutputFileFactory deleteFileFactory,Context context) {this.delegate =new BasePositionDeltaWriter<>(newInsertWriter(table, writerFactory, dataFileFactory, context),newUpdateWriter(table, writerFactory, dataFileFactory, context),newDeleteWriter(table, writerFactory, deleteFileFactory, context));this.io = table.io();this.specs = table.specs();Types.StructType partitionType = Partitioning.partitionType(table);this.deletePartitionRowWrapper = initPartitionRowWrapper(partitionType);this.deletePartitionProjections = buildPartitionProjections(partitionType, specs);this.specIdOrdinal = context.metadataSparkType().fieldIndex(MetadataColumns.SPEC_ID.name());this.partitionOrdinal =context.metadataSparkType().fieldIndex(MetadataColumns.PARTITION_COLUMN_NAME);this.fileOrdinal = context.deleteSparkType().fieldIndex(MetadataColumns.FILE_PATH.name());this.positionOrdinal =context.deleteSparkType().fieldIndex(MetadataColumns.ROW_POSITION.name());}@Overridepublic void delete(InternalRow meta, InternalRow id) throws IOException {int specId = meta.getInt(specIdOrdinal);PartitionSpec spec = specs.get(specId);InternalRow partition = meta.getStruct(partitionOrdinal, deletePartitionRowWrapper.size());// 得到指定的specId对应的分区投影器StructProjection partitionProjection = deletePartitionProjections.get(specId);// 通过分区字段的投影器,解析数据行对应的字段值partitionProjection.wrap(deletePartitionRowWrapper.wrap(partition));// 被删除的数据记录所在的文件路径String file = id.getString(fileOrdinal);// 被删除的数据记录在文件中的位置(行号)long position = id.getLong(positionOrdinal);// 最终会调用ClusteredPositionDeleteWriter::delete(…)方法,写出到position delete 文件,// 写出信息,(file, position, partitionProject),会被封装成一个PositionDelete实例,写出到position delete文件,// 实际上文件中的一行,因此position delete文件包含的数据行的结构也就很明显了// 注意这里的position delete file的数据格式,与Flink模块中的的writer的实现PartitionedDeltaWriter是不同的delegate.delete(file, position, spec, partitionProjection);}@Overridepublic WriterCommitMessage commit() throws IOException {close();// public class WriteResult implements Serializable {// private DataFile[] dataFiles;// private DeleteFile[] deleteFiles;// private CharSequence[] referencedDataFiles;// }WriteResult result = delegate.result();return new DeltaTaskCommit(result);}@Overridepublic void abort() throws IOException {close();WriteResult result = delegate.result();cleanFiles(io, Arrays.asList(result.dataFiles()));cleanFiles(io, Arrays.asList(result.deleteFiles()));}@Overridepublic void close() throws IOException {if (!closed) {delegate.close();this.closed = true;}}private PartitioningWriter<InternalRow, DataWriteResult> newInsertWriter(Table table,SparkFileWriterFactory writerFactory,OutputFileFactory fileFactory,Context context) {long targetFileSize = context.targetDataFileSize();if (table.spec().isPartitioned() && context.fanoutWriterEnabled()) {return new FanoutDataWriter<>(writerFactory, fileFactory, table.io(), targetFileSize);} else {return new ClusteredDataWriter<>(writerFactory, fileFactory, table.io(), targetFileSize);}}private PartitioningWriter<InternalRow, DataWriteResult> newUpdateWriter(Table table,SparkFileWriterFactory writerFactory,OutputFileFactory fileFactory,Context context) {long targetFileSize = context.targetDataFileSize();if (table.spec().isPartitioned()) {// use a fanout writer for partitioned tables to write updates as they may be out of orderreturn new FanoutDataWriter<>(writerFactory, fileFactory, table.io(), targetFileSize);} else {return new ClusteredDataWriter<>(writerFactory, fileFactory, table.io(), targetFileSize);}}private ClusteredPositionDeleteWriter<InternalRow> newDeleteWriter(Table table,SparkFileWriterFactory writerFactory,OutputFileFactory fileFactory,Context context) {long targetFileSize = context.targetDeleteFileSize();return new ClusteredPositionDeleteWriter<>(writerFactory, fileFactory, table.io(), targetFileSize);}
}
相关文章:

Iceberg基于Spark MergeInto语法实现数据的增量写入
SPARK SQL 基本语法 示例SQL如下 MERGE INTO target_table t USING source_table s ON s.id t.id //这里是JOIN的关联条件 WHEN MATCHED AND s.opType delete THEN DELETE // WHEN条件是对当前行进行打标的匹配条件 WHEN MATCHED AND s.opType update THEN…...

JavaScript Array(数组) 对象
JavaScript 中的 Array(数组)对象是一种用来存储一系列值的容器,它可以包含任意类型的数据,包括数字、字符串、对象等等。通过使用数组对象,我们可以轻松地组织和处理数据,以及进行各种操作,比如…...

Debian如何更换apt源
中科大 deb https://mirrors.ustc.edu.cn/debian/ stretch main non-free contrib deb https://mirrors.ustc.edu.cn/debian/ stretch-updates main non-free contrib deb https://mirrors.ustc.edu.cn/debian/ stretch-backports main non-free contrib deb-src https://mirr…...

Connext DDSPersistence Service持久性服务
DDS持久性服务,它保存了DDS数据样本,以便即使发布应用程序已经终止,也可以稍后将其发送到加入系统的订阅应用程序。 简介Persistence Service是一个Connext DDS应用程序,它将DDS数据样本保存到临时或永久存储中,因此即使发布应用程序已经终止,也可以稍后将其交付给加入系…...
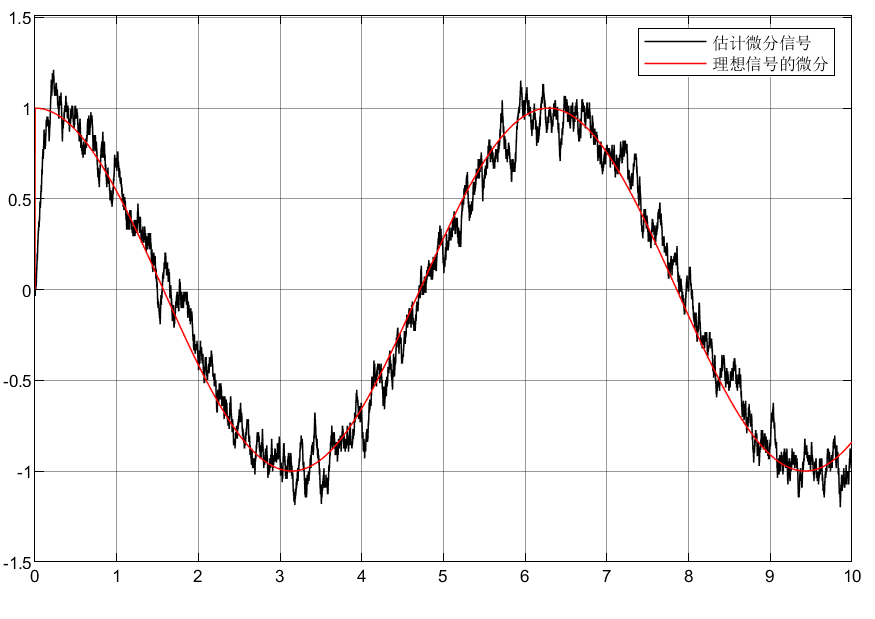
自抗扰控制ADRC之微分器TD
目录 前言 1 全程快速微分器 1.1仿真分析 1.2仿真模型 1.3仿真结果 1.4结论 2 Levant微分器 2.1仿真分析 2.2仿真模型 2.3仿真结果 3.总结 前言 工程上信号的微分是难以得到的,所以本文采用微分器实现带有噪声的信号及其微分信号提取,从而实现…...

链表学习之复制含随机指针的链表
链表解题技巧 额外的数据结构(哈希表);快慢指针;虚拟头节点; 复制含随机指针的链表 该链表节点的结构如下: class ListRandomNode { public:ListRandomNode() : val(0), next(nullptr), random(nullptr…...
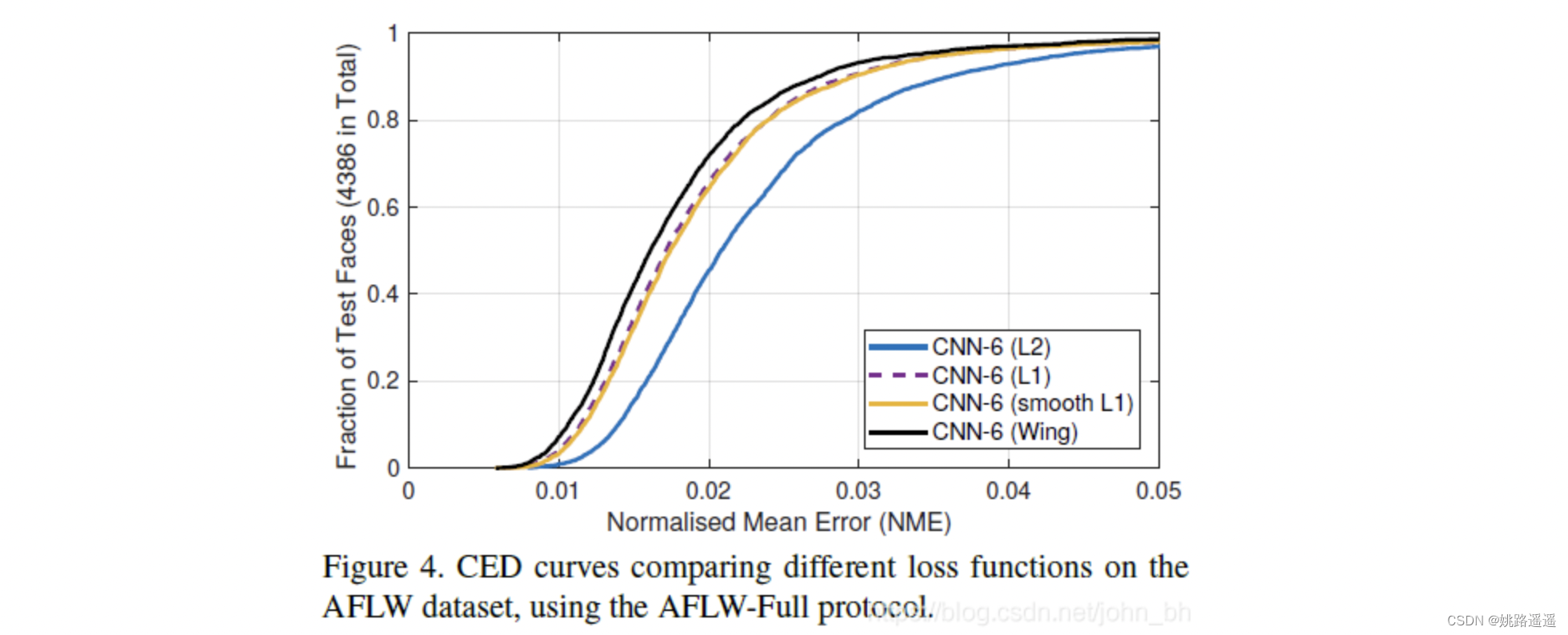
【人脸检测】Yolov5Face:优秀的one-stage人脸检测算法
论文题目:《YOLO5Face: Why Reinventing a Face Detector》 论文地址:https://arxiv.org/pdf/2105.12931.pdf 代码地址:https://github.com/deepcam-cn/yolov5-face 1.简介 近年来,CNN在人脸检测方面已经得到广泛的应用。但是许多…...
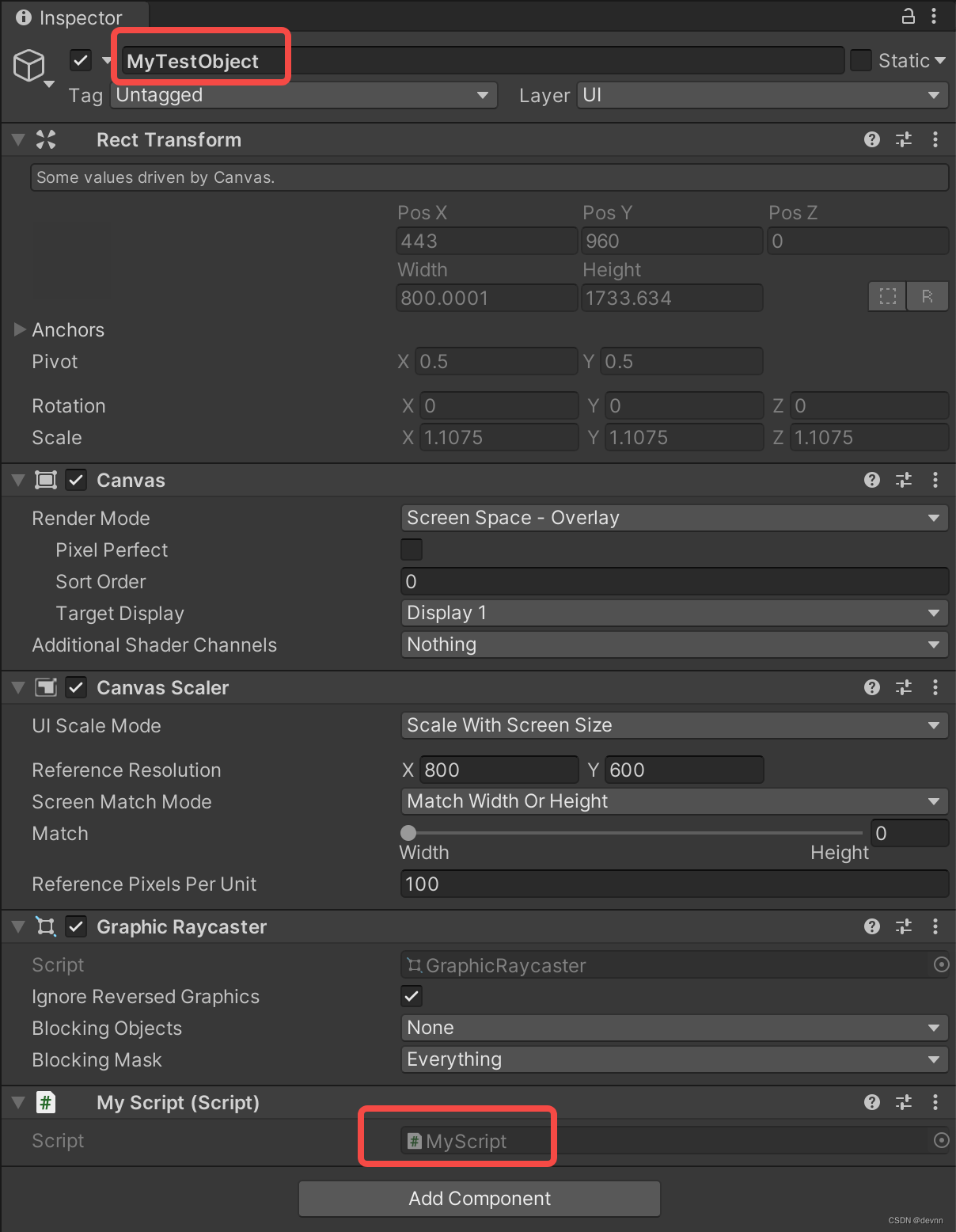
【Unity3d】Unity与Android之间通信
在unity开发或者sdk开发经常遇到unity与移动端原生层之间进行通信,这里把它们之间通信做一个整理。 关于Unity与iOS之间通信,参考【Unity3d】Unity与iOS之间通信 Unity(c#)调用Android (一)、编写Java代码 实际上,任何已经存在的Java代码…...
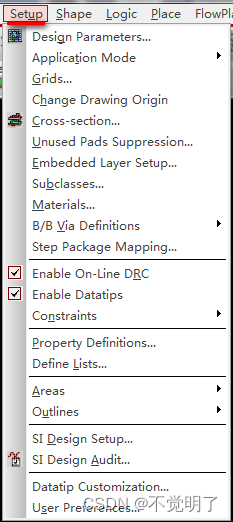
Allegro如何更改DRC尺寸大小操作指导
Allegro如何更改DRC尺寸大小操作指导 在做PCB设计的时候,DRC可以辅助设计,有的时候DRC的尺寸过大会影响视觉,Allegro支持将DRC的尺寸变小或者改大 如下图,DRC尺寸过大 如何改小,具体操作如下 点击Setup选择Design Parameters...

Mongodb WT_PANIC: WiredTiger library panic
文章目录故障现象排查过程1.查看Log2.同步恢复数据故障现象 周五突然收到Mongo实例莫名奇妙挂了告警,一般都是RS复制集架构模式(5节点),查看此实例角色为SECONDAR,挂了暂时不影响线上业务,但还是需要尽快修…...
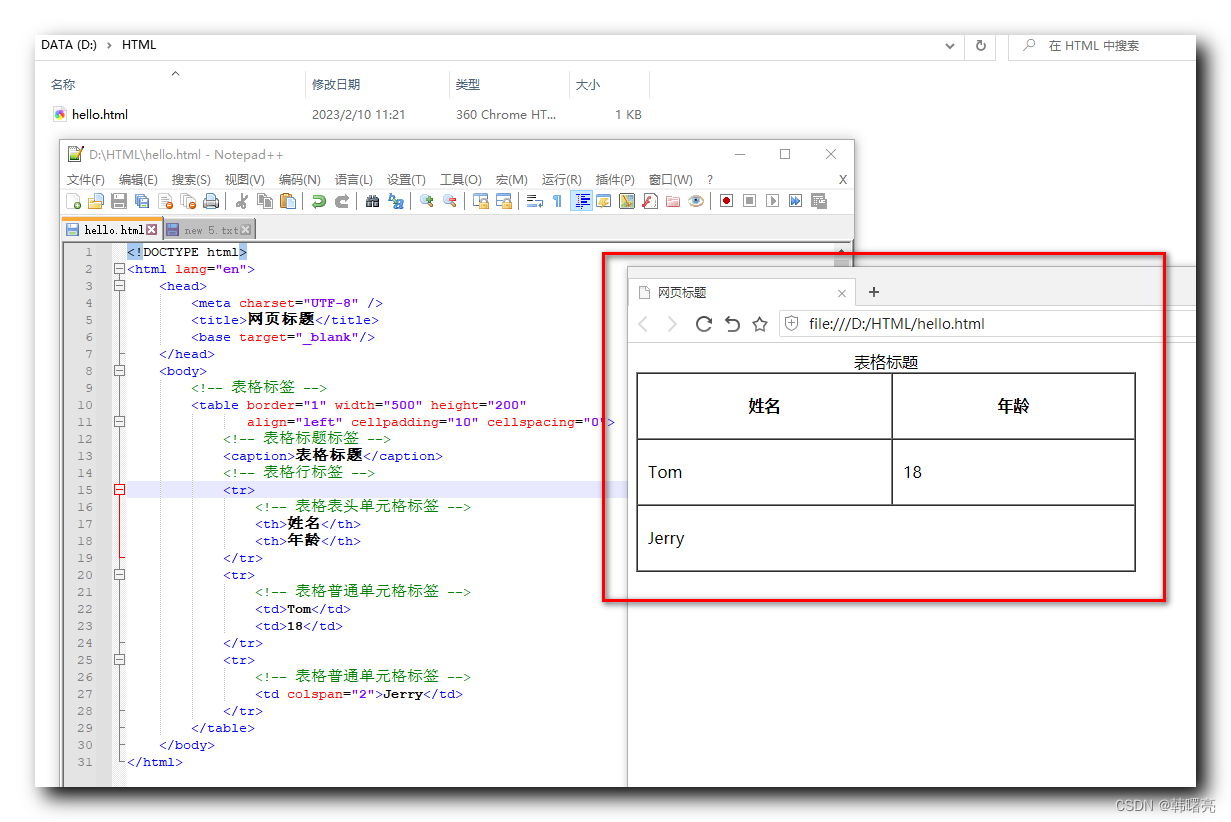
【HTML】HTML 表格总结 ★★★ ( 表格标签 | 行标签 | 单元格标签 | 表格标签属性 | 表头单元格标签 | 表格标题标签 | 合并单元格 )
文章目录一、表格标签组成 ( 表格标签 | 行标签 | 单元格标签 )二、table 表格属性 ( border 属性 | align 属性 | width 属性 | height 属性 )三、表头单元格标签四、表格标题标签五、合并单元格1、合并单元格方式2、合并单元格顺序3、合并单元格流程六、合并单元格示例1、原始…...

linux013之文件和目录的权限管理
用户、组、文件目录的关系: 简介:用户和组关联,组合文件目录关联,这样就实现了用户对文件的权限管理。首先来看一下,一个文件或目录的权限是怎么查看的,ls -l, 如下,这个信息怎么看呢…...
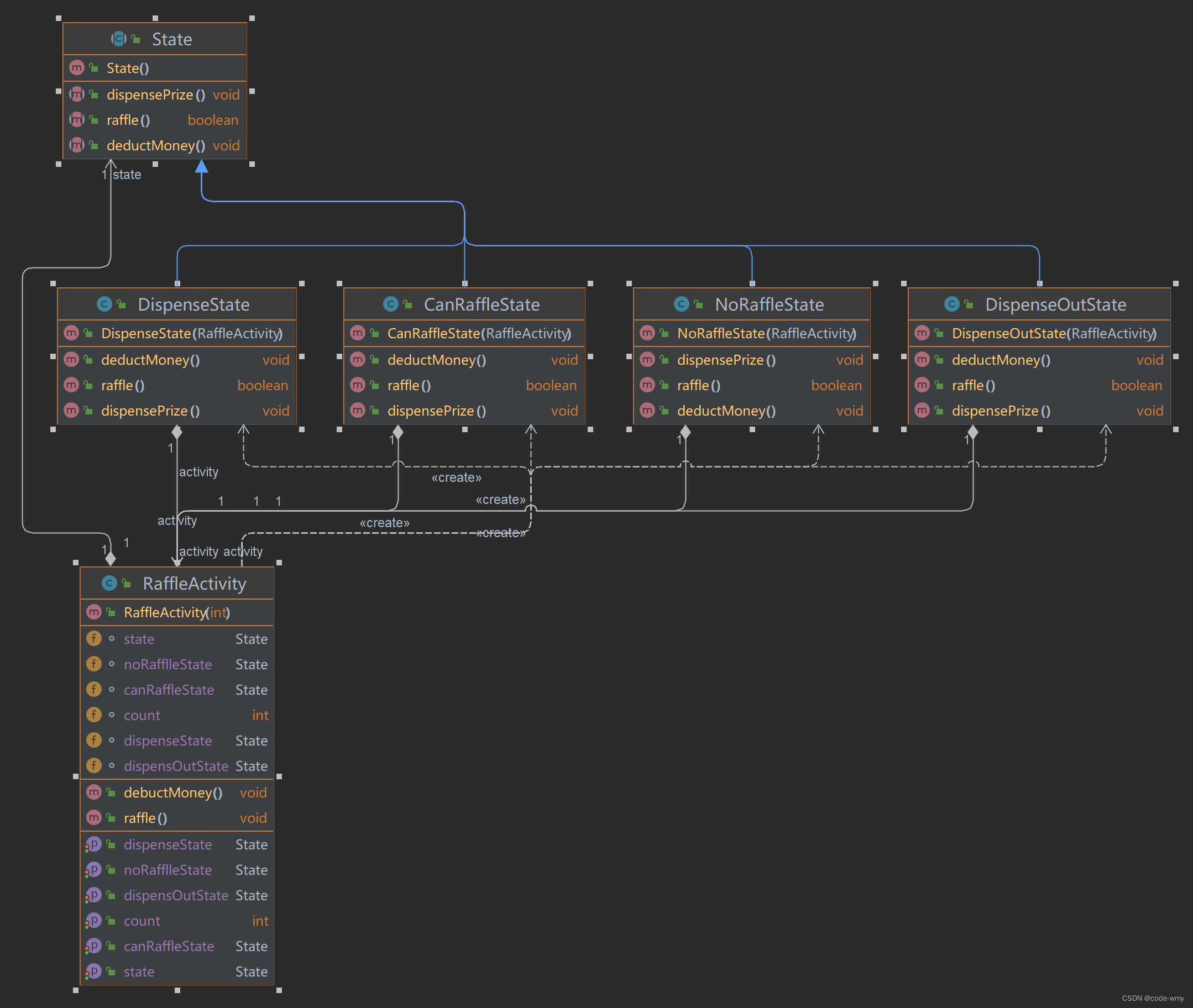
设计模式之状态模式
什么是状态模式 状态模式是指允许一个对象在其内部状态改变时改变他的行为,对象看起来似乎改变了整个类。 状态模式将一个对象在不同状态下的不同行为封装在一个个状态类中,通过设置不同的状态对象可以让环境对象拥有不同的行为,而状…...

XQuery 选择 和 过滤
XML实例文档 我们将在下面的例子中继续使用这个 "books.xml" 文档(和上面的章节所使用的 XML 文件相同)。 在您的浏览器中查看 "books.xml" 文件。 选择和过滤元素 正如在前面的章节所看到的,我们使用路径表达式或 FL…...

室友打了一把王者的时间,我理清楚了grep,find,管道|,xargs的区别与联系,用的时候不知道为什么要这样用
目录 问题引入 find和grep的基本区别 xargs命令 Linux命令的标准输入 vs 命令行参数 举例总结 问题引入 在自己做项目的过程中,想使用linux命令统计下一个目录下html文件的数量,在思考应该使用grep还是find去配合wc指令统计文件数量,后来…...
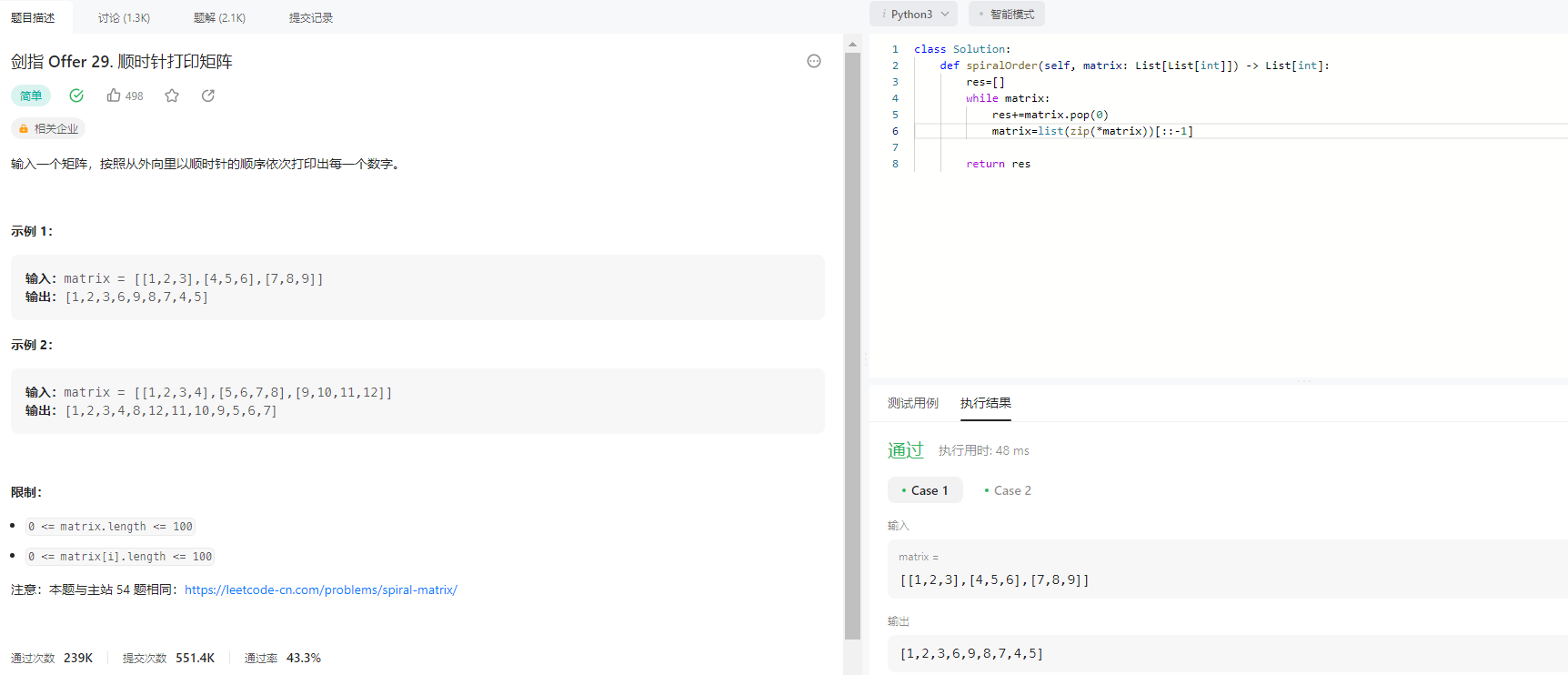
python 刷题时常见的函数
collections.OrderedDict 1. move_to_end() move_to_end() 函数可以将指定的键值对移动到最前面或者最后面,即最左边或最右边 。 2. popitem() popitem()可以完成元素的删除操作,有一个可选参数last(默认为True),…...

Python之列表推导式和列表排序
Python中的列表推导式,是小编比较喜欢的一种,他能大大减少你的代码量来得到你想要的结果,下面说说列表中常用的几种推导式 列表排序 Python开发中会经常用到排序操作,这里提供两种方式供大家参考,对象的sort()方法和…...
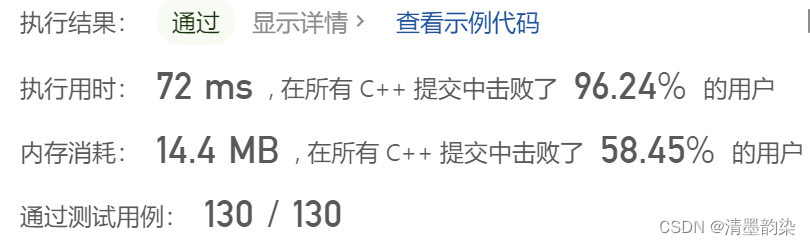
力扣(LeetCode)240. 搜索二维矩阵 II(C++)
题目描述 枚举 枚举整个矩阵,找到等于 target 的元素,则 return true ,否则 return false。 class Solution { public:bool searchMatrix(vector<vector<int>>& matrix, int target) {int n matrix.size(), m matrix[0]…...

golang defer
文章目录延迟函数的参数在defer语句出现时就已经确定下来了延迟函数没有入参时,延迟函数体内的变量会受到影响延迟函数 *可以* 修改主函数的 *具名* 返回值延迟函数 *无法* 修改主函数的 *匿名* 返回值defer会把声明的 延迟函数以及 函数的入参放到栈上,…...
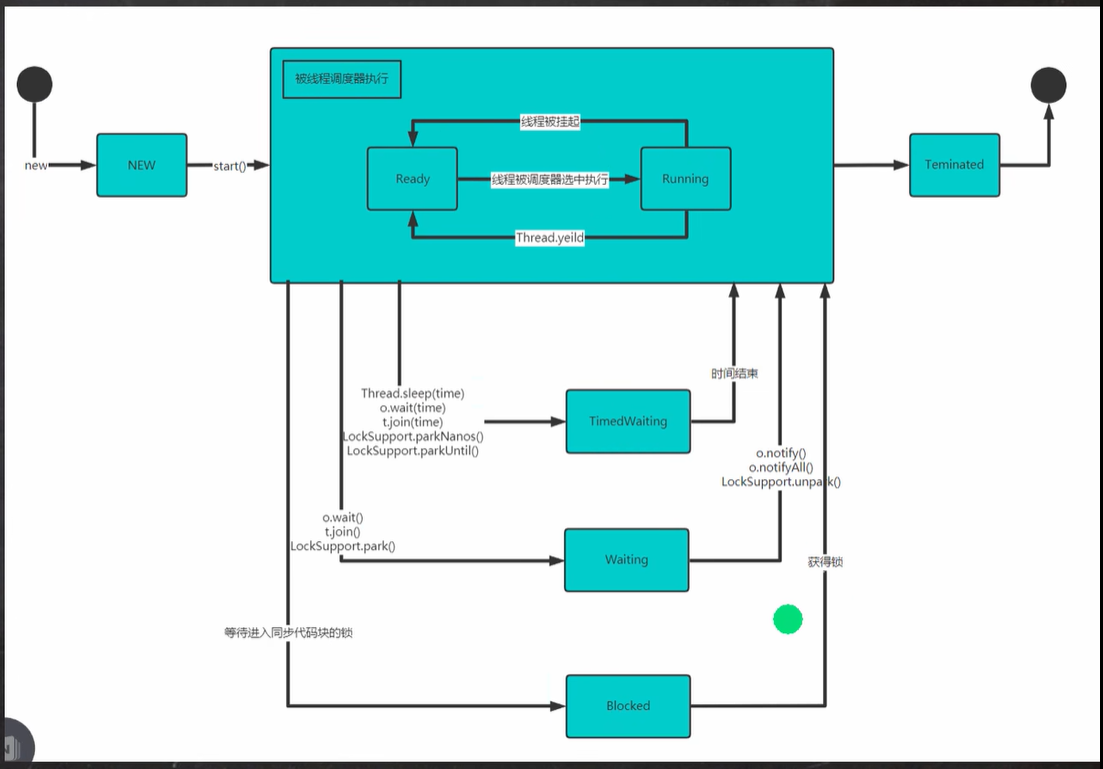
【Java】线程的死锁和释放锁
线程死锁是线程同步的时候可能出现的一种问题 文章目录1. 线程的死锁1.1 基本介绍1.2 应用案例2. 释放锁2.1 下面的操作会释放锁2.2 下面的操作不会释放锁1. 线程的死锁 1.1 基本介绍 多个线程都占用了对方的锁资源,但不肯相让,导致了死锁,…...
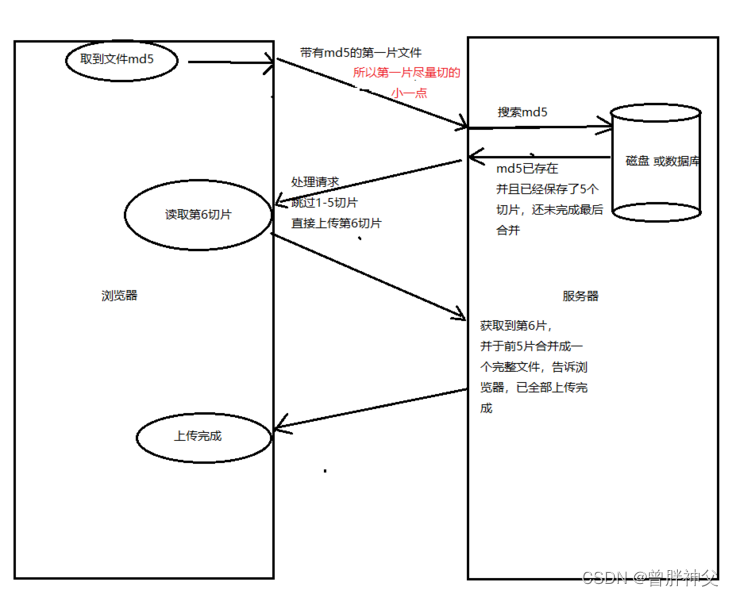
如何使用断点续传上传大文件
概念 大文件上传的需求介绍 不管怎样简单的需求,在量级达到一定层次时,都会变得异常复杂。 文件上传简单,文件变大就复杂 上传大文件时,以下几个变量会影响我们的用户体验 服务器处理数据的能力请求超时网络波动 上传时间会变长…...

【图神经网络】图拉普拉斯滤波器如何实现全通、低通、高通滤波
【图神经网络】图拉普拉斯滤波器如何实现全通、低通、高通滤波 文章目录【图神经网络】图拉普拉斯滤波器如何实现全通、低通、高通滤波1. 前言2. 符号说明3. 三种滤波3.1 全通滤波3.2 低通滤波3.2.1 平滑信号分析3.2.2 广义拉普拉斯平滑滤波器3.3 高通滤波4. 总结1. 前言 GCN&…...

python操作mysql数据库详解
使用Python操作MySQL数据库 MySQL是一种关系型数据库管理系统,它可以用来存储和管理大量的数据。之前介绍了大部分主流数据库,今天将介绍如何使用Python来操作MySQL数据库。 安装MySQL 首先,我们需要安装MySQL服务器,可以从MyS…...
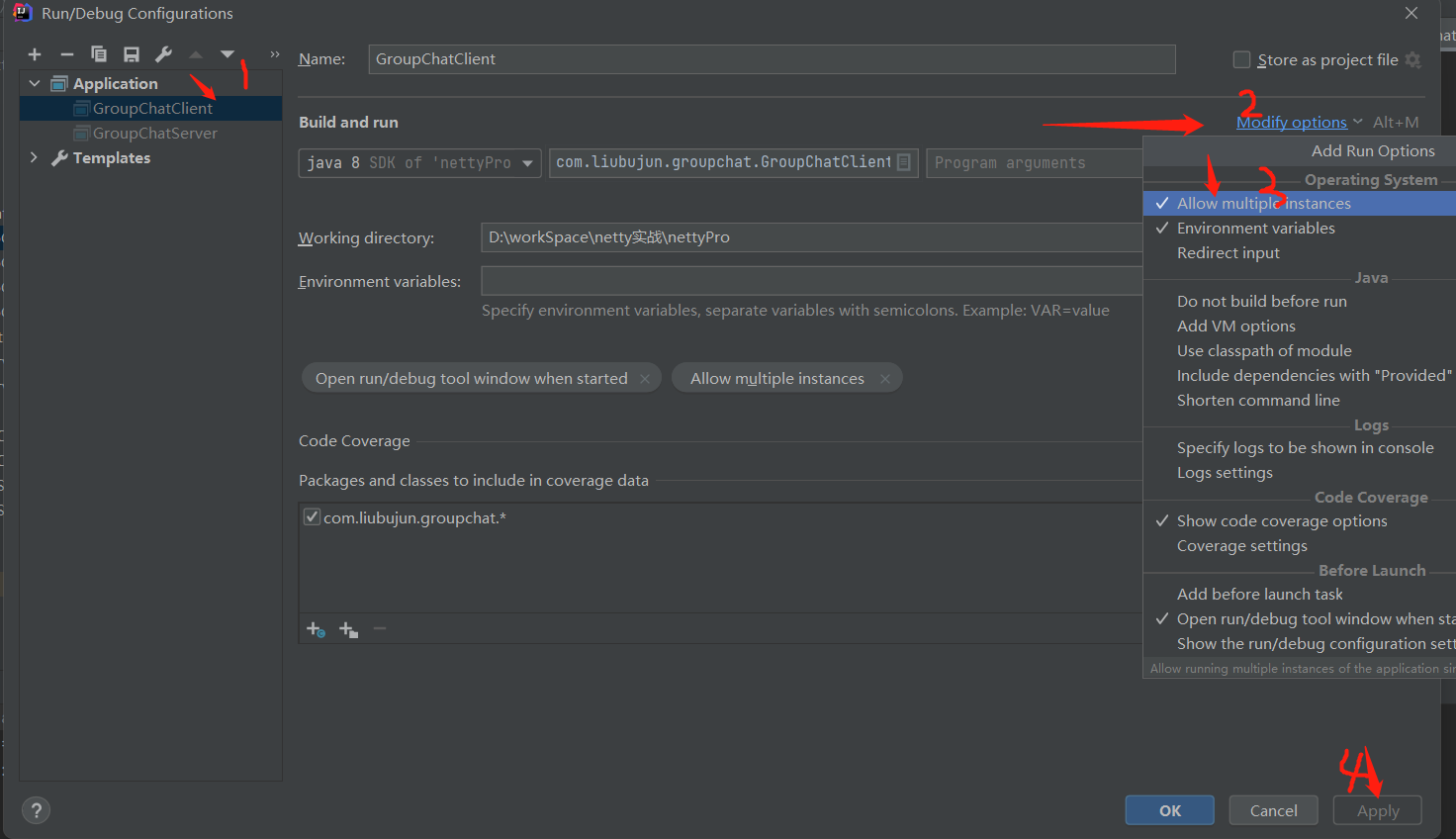
netty群聊系统
1设计思路:启动一个服务端,多个客户端第一个客户端启动时,会告诉服务器上线了第二个客户端启动时,告诉服务器上线,并且通知第一个启动的客户端第三个客户端启动时,告诉服务器上线,并且通知第一个…...
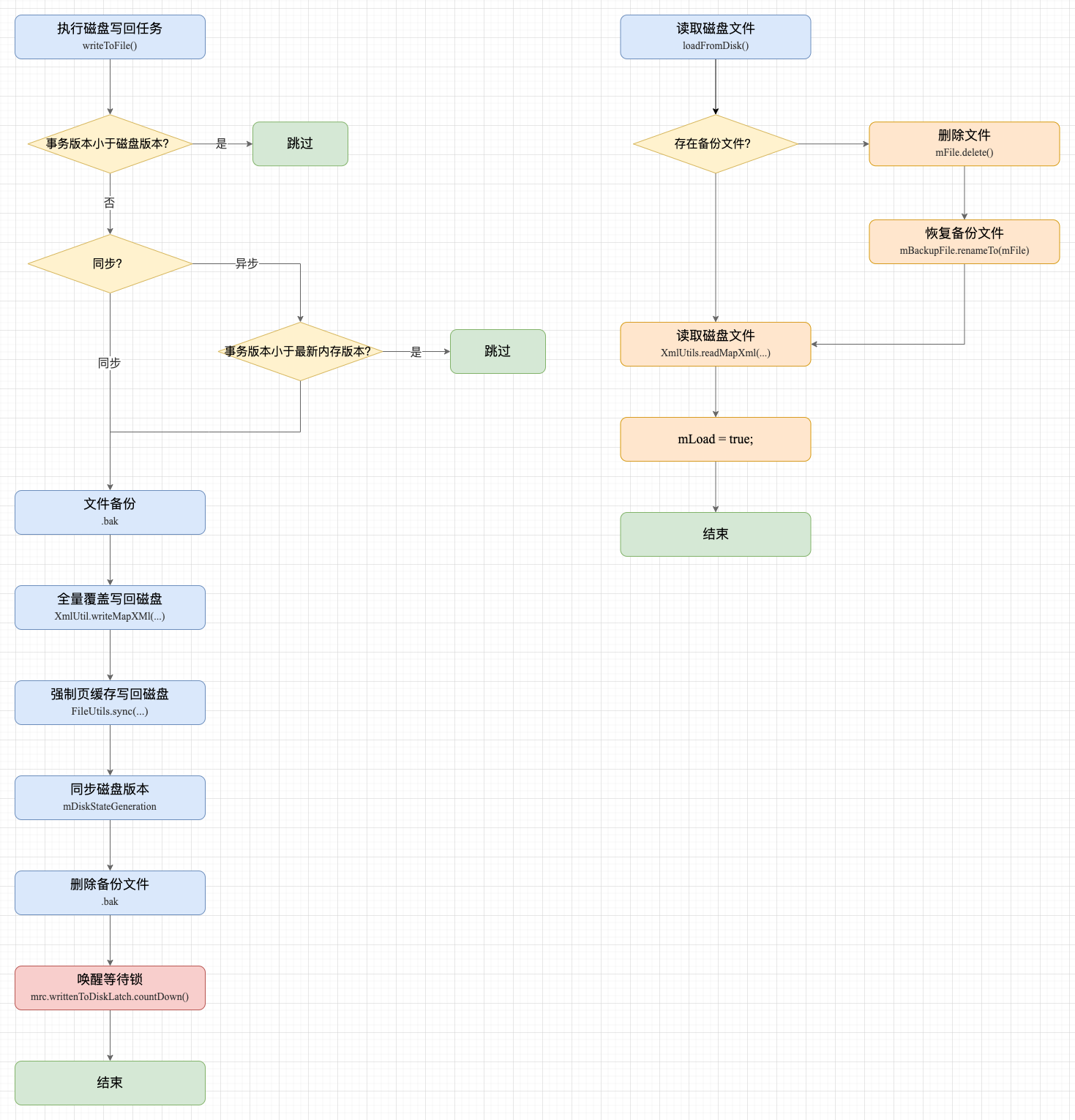
Android 初代 K-V 存储框架 SharedPreferences,旧时代的余晖?
本文已收录到 AndroidFamily,技术和职场问题,请关注公众号 [彭旭锐] 提问。 前言 大家好,我是小彭。 SharedPreferences 是 Android 平台上轻量级的 K-V 存储框架,亦是初代 K-V 存储框架,至今被很多应用沿用。 有的…...
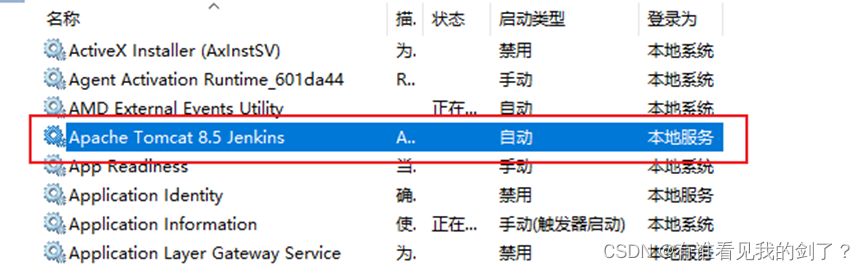
在windows中使用tomcat搭建Jenkins
1、 准备环境:JDK JDK官网下载:https://download.oracle.com/java/19/latest/jdk-19_windows-x64_bin.msi 2、 tomcat包 tocat官网下载:https://tomcat.apache.org/download-90.cgi 3、 Jenkins.war包 Jenkins官网下载:https://mi…...

Linux系统
linux系统 世界上最重要的服务器端操作系统。 创建新目录 mkdir app mkdir -m 目录权限 目录名 创建有权限的目录名。 创建一个空白文件 touch app.txt创建一个文件。 cat创建一个文件。 vi/vim创建一个文件。 nano创建一个文件。 truncate创建一个文件。 pwd查看当前目录。 rm…...
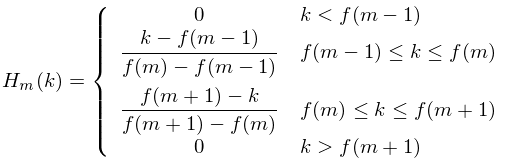
Mel Frequency Cepstral Coefficients (MFCCs)
wiki里说 在声音处理中,梅尔频率倒谱( MFC ) 是声音的短期功率谱的表示,基于非线性梅尔频率标度上的对数功率谱的线性余弦变换。 倒谱和MFC 之间的区别在于,在 MFC 中,频带在梅尔尺度上等距分布,这比正常频谱中使用的线…...
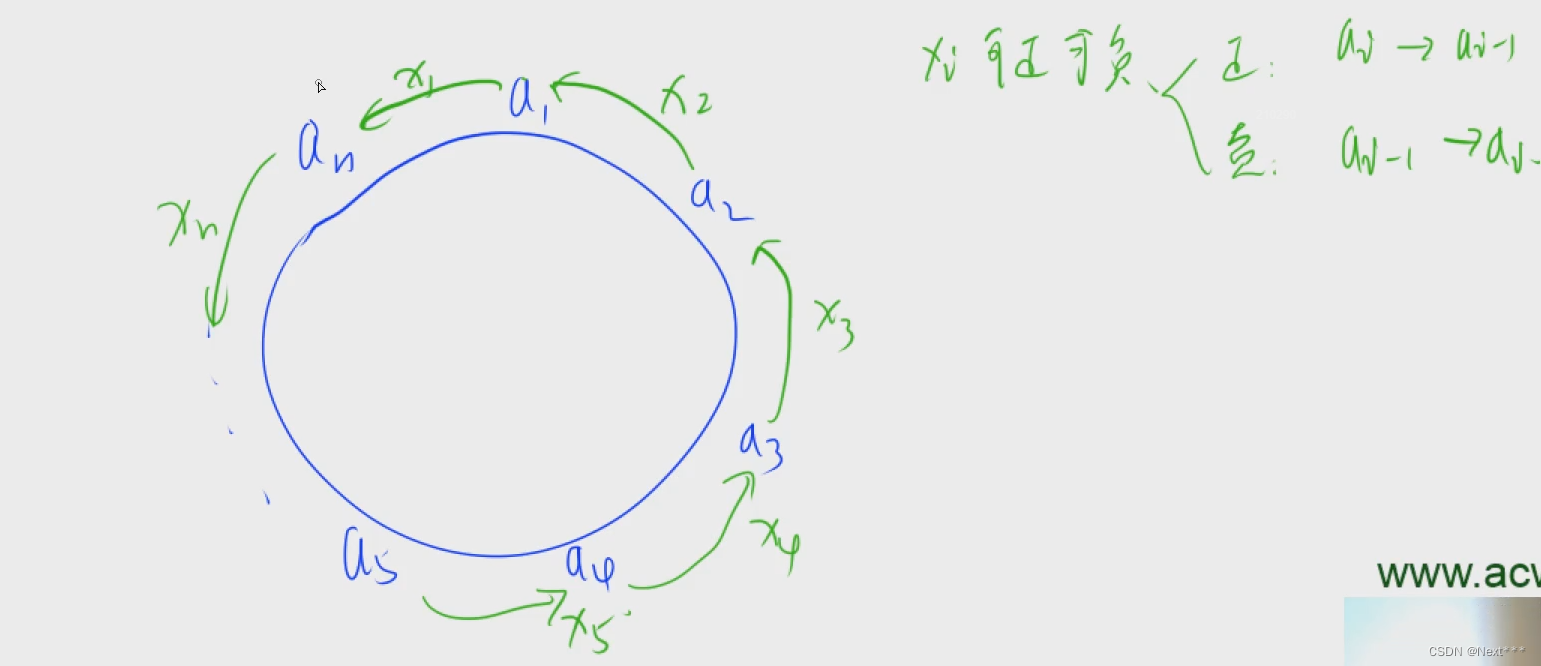
第七讲---贪心(上课)
1.股票买卖 一、贪心 考虑一种方案,在每次上升的前一天购入股票,并在上升后的当天卖出的方案 if (w[i] > w[i - 1])res w[i] - w[i - 1];接下来证明该贪心思路得出的方案即是最优解。 (1)证明贪心解 ≥ 最优解: …...

计算机如何思考与图灵完备
图灵完备是针对一套数据操作规则而言的概念,数据操作规则可以是一门编程语言,也可以是计算机实现里面的指令集,比如C/C++是图图灵完备的,通用CPU也是图灵完备的,但是GPU却不一定是图灵完备的。说白了图灵完备定义了一套规则,当这套规则可以实现图灵迹模型里的全部功能时,…...