C++11使用多线程(线程池)计算相似度实现性能优化
需求:图像识别中,注册的样本多了会影响计算速度,成为性能瓶颈,其中一个优化方法就是使用多线程。例如,注册了了3000个特征,每个特征4096个float。可以把3000个特征比对放到4个线程中进行计算,然后再把结果进行合并。实现思路:
1. 根据系统性能和需求配置线程池的大小,创建线程池,将比较任务平均分配到各个线程
2. 工作线程启动后在一个condition_variable上wait,注意:锁的范围不能太大了,否则多个线程会变成串行
3. 调用者调用识别接口,接口更新目标特征,通知各个工作线程,在另外一个condition_variable上wait,并且满足完成计数器的值等于线程数
4. 工作线程完成后将计数器加一,并且通知调用线程
5. 调用线程收集到所有线程的结果后再对结果进行合并返回
后续:
1. 代码进行优化,更优雅的实现
测试结果:
线程数 | 时间 |
1 | 71362ms |
2 | 36292ms |
4 | 19420ms |
8 | 18465ms |
16 | 18433ms |
32 | 18842ms |
64 | 19324ms |
128 | 19388ms |
256 | 21853ms |
512 | 26150ms |
1024 | 35593ms |
代码如下:
#include <iostream>
#include <string>
#include <cstring>
#include <mutex>
#include <unordered_map>
#include <list>
#include <utility>
#include <algorithm>
#include <string>
#include <vector>
#include <thread>
#include <chrono>
using namespace std;
using namespace chrono;
double get_mold(const vector<double> &vec)
{
int n = vec.size();
double sum = 0.0;
for(int i = 0; i < n; ++i)
{
sum += vec[i] * vec[i];
}
return sqrt(sum);
}
double cosine_distance(const vector<double> &base, const vector<double> &target)
{
int n = base.size();
double tmp = 0.0;
for(int i = 0; i < n; ++i)
{
tmp += base[i] * target[i];
}
double simility = tmp / (get_mold(base) * get_mold(target));
return simility;
}
class Recognizer
{
public:
Recognizer(int num_threads) :
num_threads_(num_threads),
is_run_calculate_thread_(true),
is_doing_recognize(false),
result_count(0)
{
recognize_result = std::vector<RecognizeResult>(num_threads);
this->load_feature();
this->init_threads();
}
~Recognizer()
{
is_run_calculate_thread_ = false;
cv_.notify_all();
for(std::thread &th : threads_)
th.join();
}
int do_recognize(const vector<double> &feature);
private:
class CigaretteItem
{
public:
int cigarette_id_;
std::string cigarette_name_;
std::vector<double> feature_;
CigaretteItem(int cigarette_id, std::string cigarette_name, const std::vector<double> &cigarette_feature)
{
cigarette_id_ = cigarette_id;
cigarette_name_ = cigarette_name;
feature_ = std::vector<double>(cigarette_feature.size());
for(int i = 0; i < cigarette_feature.size(); i++)
{
feature_[i] = cigarette_feature[i];
}
}
};
class RecognizeResult
{
public:
int cigarette_id_;
std::string cigarette_name_;
double score_;
};
private:
int num_threads_;
bool is_run_calculate_thread_;
bool is_doing_recognize;
std::vector<CigaretteItem> ciagarette_list_;
std::vector<double> target_feature_;
std::mutex cv_mtx_;
std::condition_variable cv_;
std::vector<RecognizeResult> recognize_result;
std::vector<std::thread> threads_;
int result_count;
std::mutex result_count_mtx_;
std::mutex result_cv_mtx_;
std::condition_variable result_cv_;
private:
Recognizer(const Recognizer&) = delete;
Recognizer& operator=(const Recognizer&) = delete;
void load_feature();
void init_threads();
void calculate_most_similarity(const int thread_id, const int start_index, const int end_index);
};
void Recognizer::load_feature()
{
for(int i = 0; i < 3000; i++)
{
vector<double> fea = vector<double>(4096);
for(int i = 0; i < 4096; ++i)
fea[i] = (double)(rand() % 998 + 1) / 1000.00;
ciagarette_list_.emplace_back(i+1, "cigarette", fea);
}
}
void Recognizer::init_threads()
{
for(int i = 0; i < num_threads_; i++)
{
int step = this->ciagarette_list_.size() / this->num_threads_;
int start_index = i * step;
int end_index = (i+1) * step;
if(i == num_threads_ - 1){
end_index = ciagarette_list_.size();
}
std::cout << "thread" << i << " starts at " << start_index << "; ends at " << end_index << std::endl;
threads_.emplace_back(&Recognizer::calculate_most_similarity, this, i, start_index, end_index);
}
}
void Recognizer::calculate_most_similarity(const int thread_id, const int start_index, const int end_index)
{
while(is_run_calculate_thread_)
{
{
std::unique_lock<std::mutex> lock(cv_mtx_);
cv_.wait(lock);
}
//cout << "thread" << thread_id << " is running" << endl;
double max_score = -1.00;
int max_score_index = -1;
for(int i = start_index; i < end_index; ++i){
double score = cosine_distance(ciagarette_list_[i].feature_, target_feature_);
if(score > max_score)
{
max_score = score;
max_score_index = i;
}
}
recognize_result[thread_id].cigarette_id_ = ciagarette_list_[max_score_index].cigarette_id_;
recognize_result[thread_id].cigarette_name_ = ciagarette_list_[max_score_index].cigarette_name_;
recognize_result[thread_id].score_ = max_score;
{
std::unique_lock<std::mutex> lock(result_count_mtx_);
result_count += 1;
}
result_cv_.notify_one();
//std::cout << "thread" << thread_id << " finish one task" << endl;
}
//std::cout << "thread" << thread_id << " finished." << std::endl;
}
int Recognizer::do_recognize(const vector<double> &feature)
{
if(is_doing_recognize)
return -1;
is_doing_recognize = true;
this->target_feature_ = feature;
//cout << "cv_.notify_all()" << endl;
cv_.notify_all();
std::unique_lock<std::mutex> lock(result_cv_mtx_);
result_cv_.wait(lock, [this](){return this->num_threads_ == this->result_count;});
//std::cout << "all threads finish computing similarity" << endl;
int max_score_cigarette_id = -1;
std::string max_score_cigarette_name = "";
double max_score = -1.0;
for(int i = 0; i < num_threads_; ++i)
{
if(recognize_result[i].score_ > max_score)
{
max_score_cigarette_id = recognize_result[i].cigarette_id_;
max_score_cigarette_name = recognize_result[i].cigarette_name_;
max_score = recognize_result[i].score_;
}
}
//cout << "cigarette_id=" << max_score_cigarette_id << ", cigarette_name=" << max_score_cigarette_name << ", score=" << max_score << endl;
this->result_count = 0;
is_doing_recognize = false;
return 0;
}
int main(void)
{
Recognizer recognizer{1024};
std::this_thread::sleep_for(std::chrono::seconds(1));
const int loops = 400;
auto start_time = system_clock::now();
for(int i = 0; i < loops; i++)
{
//cout << endl;
std::vector<double> target_feature = std::vector<double>(4096);
for(int i = 0; i < 4096; ++i)
{
//target_feature[i] = (double)(rand() % 998 + 1) / 1000.000;
target_feature[i] = (double)(i % 1000 + 1) / 1000.00;
}
recognizer.do_recognize(target_feature);
//if((i+1) % 20 == 0)
// cout << "i=" << i << endl;
}
auto end_time = system_clock::now();
auto duration = duration_cast<milliseconds>(end_time - start_time);
cout << "eplased_time:" << duration.count() << "ms" << endl;
std::this_thread::sleep_for(std::chrono::seconds(2));
return 0;
}
相关文章:

C++11使用多线程(线程池)计算相似度实现性能优化
需求:图像识别中,注册的样本多了会影响计算速度,成为性能瓶颈,其中一个优化方法就是使用多线程。例如,注册了了3000个特征,每个特征4096个float。可以把3000个特征比对放到4个线程中进行计算,然…...
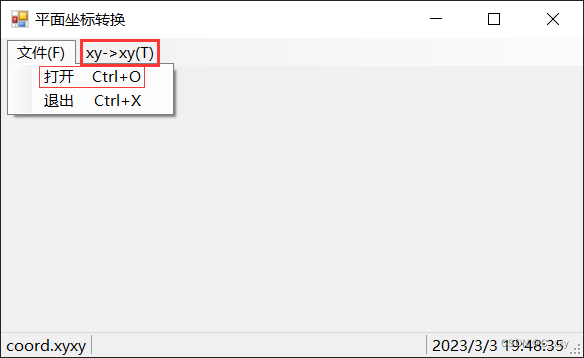
【测绘程序设计】——平面坐标转换
测绘工程中经常遇到平面坐标转换——比如,北京54(或西安80)平面坐标转换成CGCS2000平面坐标、工程独立坐标系平面坐标转换成CGCS2000平面坐标等,常用转换模型包括:①三参数法(2平移+1旋转);②四参数法(赫尔默特法,2平移+1旋转+1尺度);③六参数法(仿射变换法,2平移…...
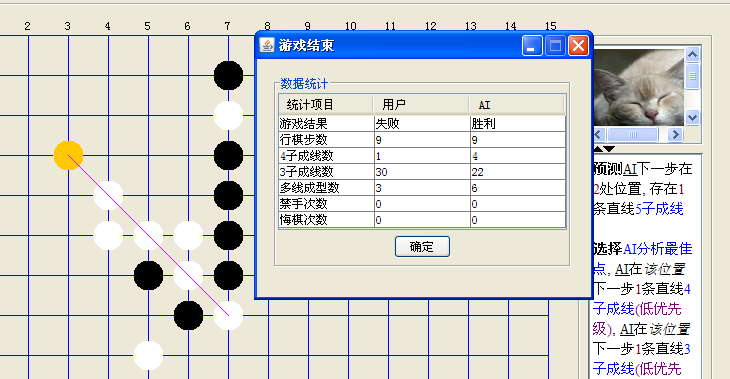
五子棋的设计与实现
术:Java等摘要:五子棋是一种两人对弈的纯策略型棋类游戏,非常容易上手,老少皆宜。为了更好的推广五子棋,研究简单的人工智能方式,运用Java开发五子棋游戏。主要包含了人机对战,棋盘初始化&#…...

大数据项目软硬件选择
目录 一.技术选型 二.系统数据流程设计 三.框架版本选型 如何选择Apache/CDH/HDP版本...
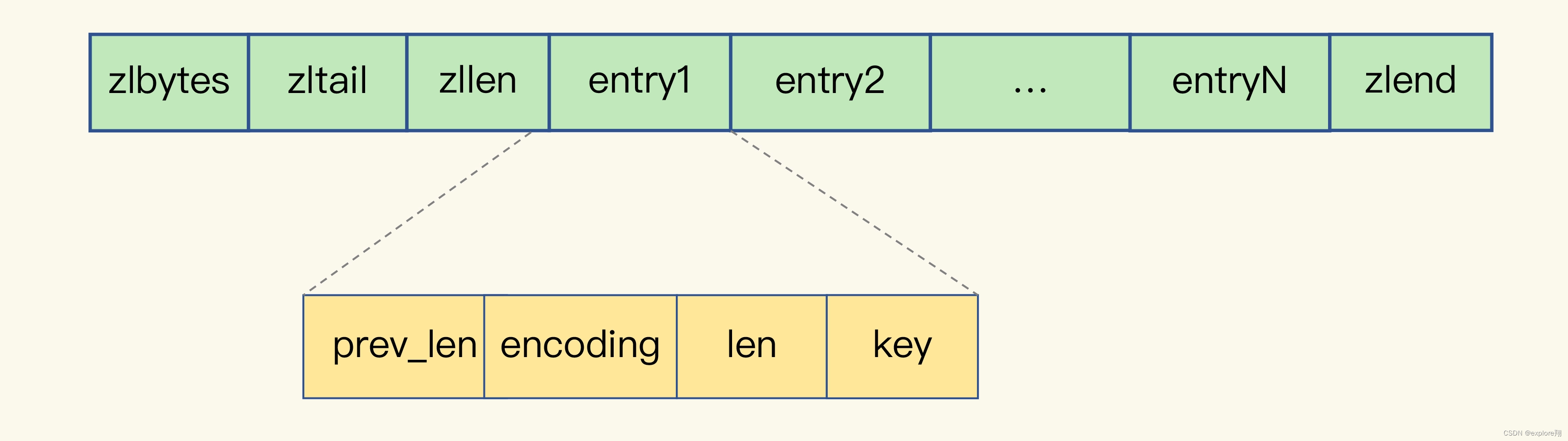
redis数据结构的适用场景分析
1、String 类型的内存空间消耗问题,以及选择节省内存开销的数据类型的解决方案。 为什么 String 类型内存开销大? 图片 ID 和图片存储对象 ID 都是 10 位数,我们可以用两个 8 字节的 Long 类型表示这两个 ID。因为 8 字节的 Long 类型最大可以…...

同步、异步、全双工、半双工的区别
1、通讯 1.1 并行通讯 定义:一条信息的各位数据被同时传送的通讯方式称为并行通讯; 特点: 各个数据位同时发送,传送速度快、效率高,但有多少数据位就需要多少根数据线,因此传送成本高,并且只…...
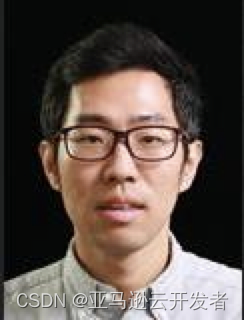
ClickHouse 与 Amazon S3 结合?一起来探索其中奥秘
目录ClickHouse 简介ClickHouse 与对象存储ClickHouse 与 S3 结合的三种方法示例参考架构小结参考资料ClickHouse 简介ClickHouse 是一种快速的、开源的、用于联机分析(OLAP)的列式数据库管理系统(DBMS),由俄罗斯的Yan…...
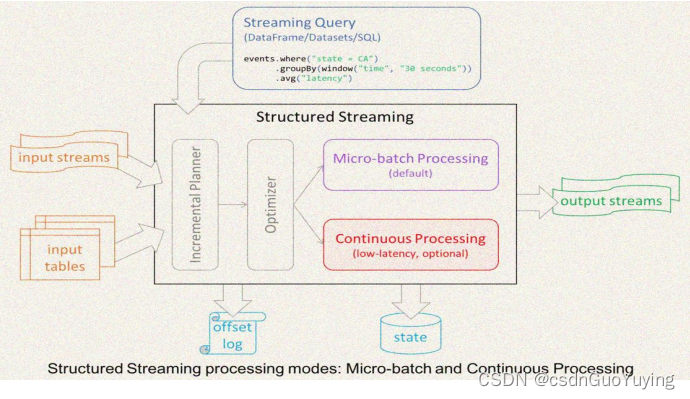
【Spark分布式内存计算框架——Structured Streaming】1. Structured Streaming 概述
前言 Apache Spark在2016年的时候启动了Structured Streaming项目,一个基于Spark SQL的全新流计算引擎Structured Streaming,让用户像编写批处理程序一样简单地编写高性能的流处理程序。 Structured Streaming并不是对Spark Streaming的简单改进…...

【Windows】【Linux】---- Java证书导入
问题: PKIX path building failed: sun.security.provider.certpath.SunCertPathBuilderException: unable to find valid certification path to requested target 无法找到请求目标的有效证书路径 一、Windows—java证书导入 1、下载证书到本地(以下…...
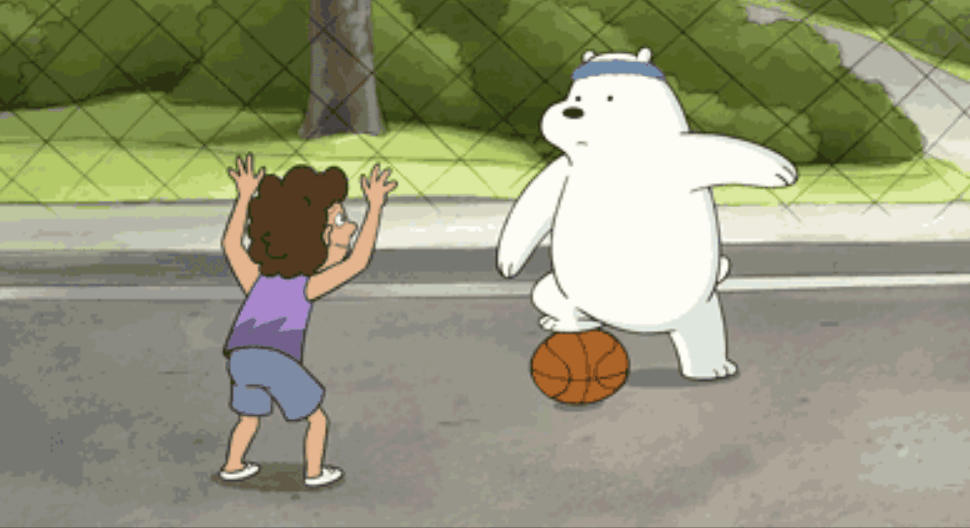
【Linux学习】菜鸟入门——gcc与g++简要使用
一、gcc/g gcc/g是编译器,gcc是GCC(GUN Compiler Collection,GUN编译器集合)中的C编译器;g是GCC中的C编译器。使用g编译文件时会自动链接STL标准库,而gcc不会自动链接STL标准库。下面简单介绍一下Linux环境下(Windows差…...

Cadence Allegro 导出Bill of Material Report详解
⏪《上一篇》 🏡《总目录》 ⏩《下一篇》 目录 1,概述2,Assigned Functions Report作用3,Assigned Functions Report示例4,Assigned Functions Report导出方法4.1,方法14.2,方法2B站关注“硬小二”浏览更多演示视频...
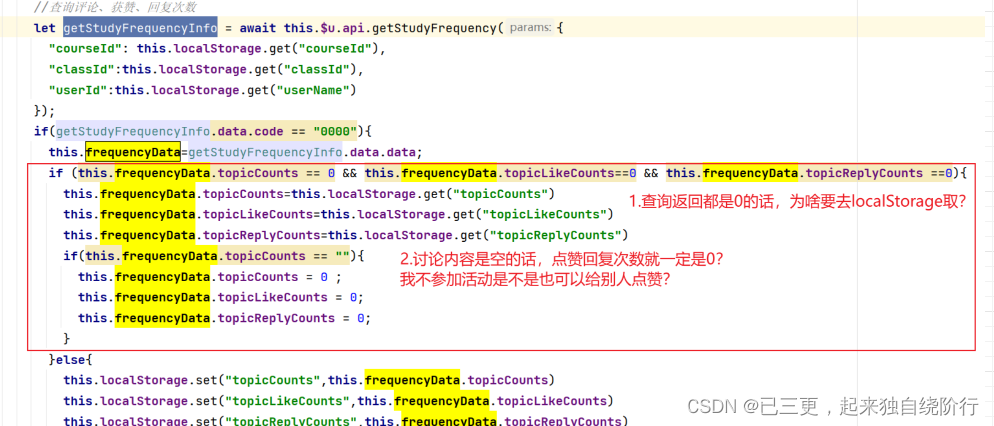
localStorage线上问题的思考
一、背景: localStorage作为HTML5 Web Storage的API之一,使用标准的键值对(Key-Value,简称KV)数据类型主要作用是本地存储。本地存储是指将数据按照键值对的方式保存在客户端计算机中,直到用户或者脚本主动清除数据&a…...

什么是DNS域名解析
什么是DNS域名解析?因特网上作为域名和IP地址相互映射的一个分布式数据库,能够使用户更方便的访问互联网,而不用去记住能够被机器直接读取的IP数串。通过主机名,得到该主机名对应的IP地址的过程叫做域名解析。正向解析:…...

Cadence Allegro 导出Assigned Functions Report详解
⏪《上一篇》 🏡《总目录》 ⏩《下一篇》 目录 1,概述2,Assigned Functions Report作用3,Assigned Functions Report示例4,Assigned Functions Report导出方法4.1,方法14.2,方法2B站关注“硬小二”浏览更多演示视频...

Python中Opencv和PIL.Image读取图片的差异对比
近日,在进行深度学习进行推理的时候,发现不管怎么样都得不出正确的结果,再仔细和正确的代码进行对比了后发现原来是Python中不同的库读取的图片数组是有差异的。 image np.array(Image.open(image_file).convert(RGB)) image cv2.imread(…...
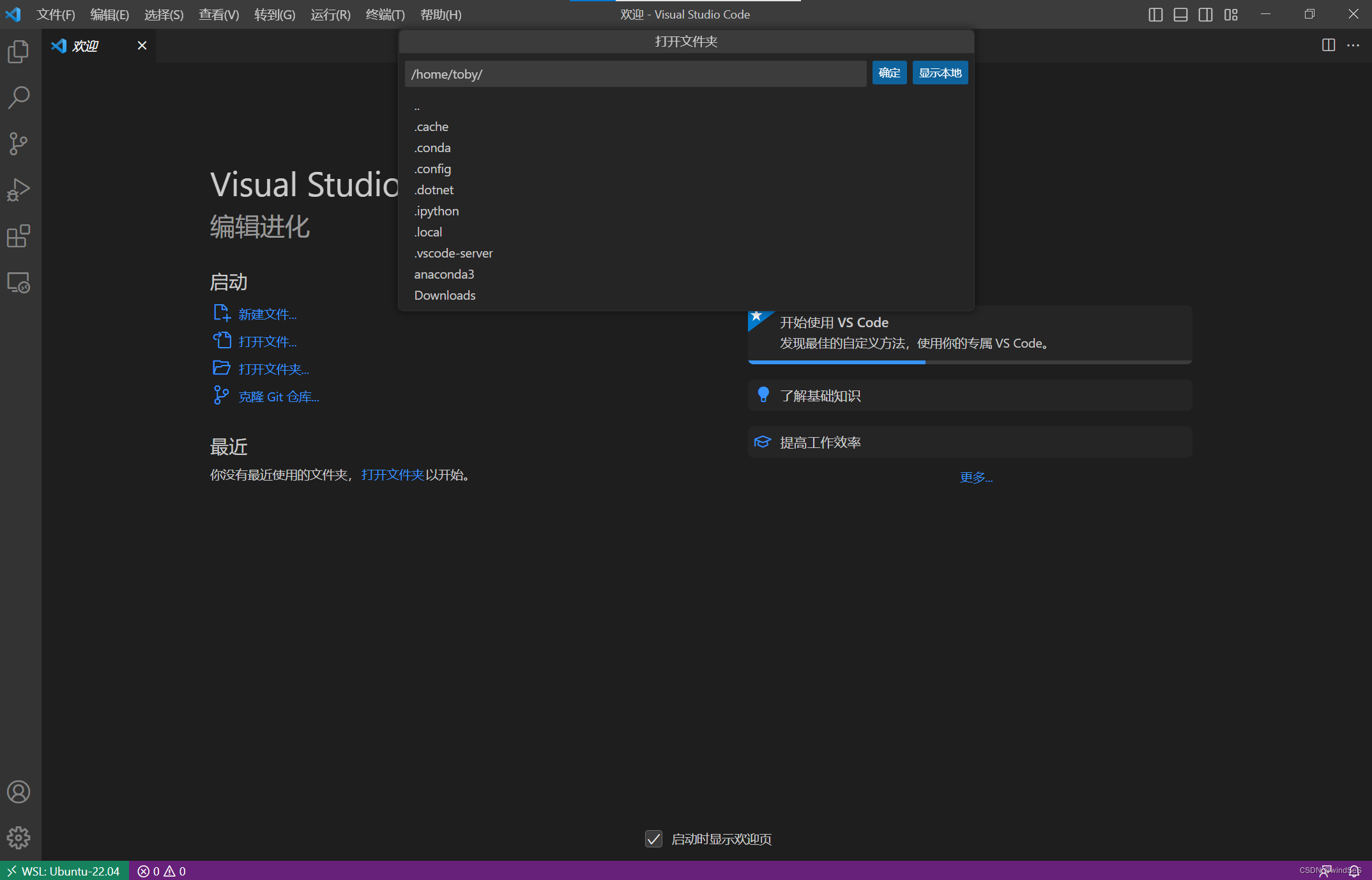
win10 WSL2 使用Ubuntu配置与安装教程
Win10 22H2ubuntu 22.04ROS2 文章目录一、什么是WSL2二、Win10 系统配置2.1 更新Windows版本2.2 Win10系统启用两个功能2.3 Win10开启BIOS/CPU开启虚拟化(VT)(很关键)2.4 下载并安装wsl_update_x64.msi2.5 PowerShell安装组件三、PowerShell安装Ubuntu3.…...

LeetCode每日一题(28. Find the Index of the First Occurrence in a String)
Given two strings needle and haystack, return the index of the first occurrence of needle in haystack, or -1 if needle is not part of haystack. Example 1: Input: haystack “sadbutsad”, needle “sad” Output: 0 Explanation: “sad” occurs at index 0 and…...
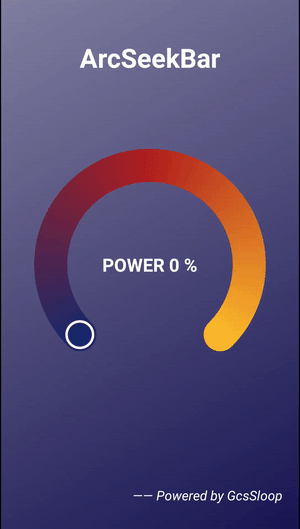
Android 圆弧形 SeekBar
效果预览package com.gcssloop.widget;import android.annotation.SuppressLint;import android.content.Context;import android.content.res.TypedArray;import android.graphics.Canvas;import android.graphics.Color;import android.graphics.Matrix;import android.graph…...
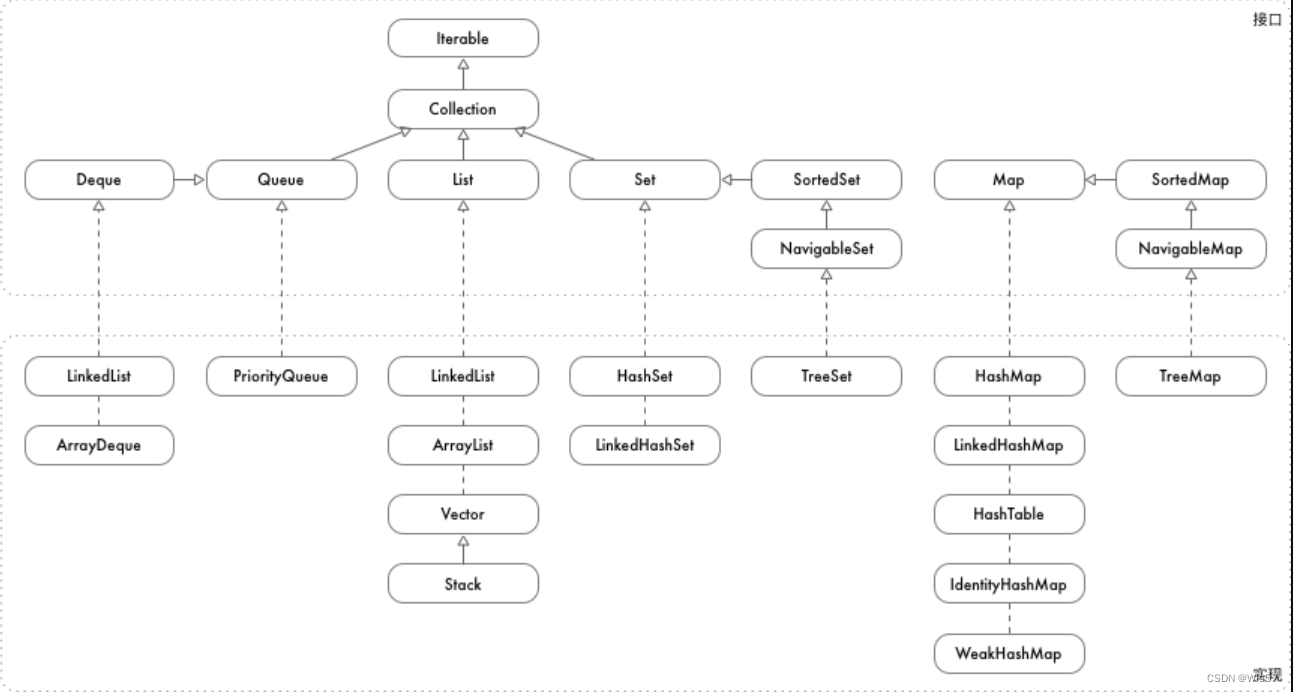
java 字典
java 字典 数据结构总览 Map Map 描述的是一种映射关系,一个 key 对应一个 value,可以添加,删除,修改和获取 key/value,util 提供了多种 Map HashMap: hash 表实现的 map,插入删除查找性能都是 O(1)&…...
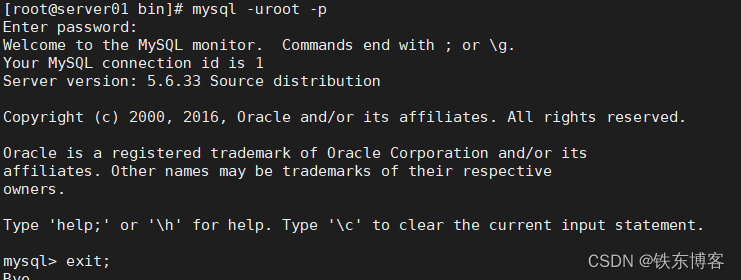
【企业服务器LNMP环境搭建】mysql安装
MySQL安装步骤: 1、相关说明 1.1、编译参数的说明 -DCMAKE_INSTALL_PREFIX安装到的软件目录-DMYSQL_DATADIR数据文件存储的路径-DSYSCONFDIR配置文件路径 (my.cnf)-DENABLED_LOCAL_INFILE1使用localmysql客户端的配置-DWITH_PARTITION_STORAGE_ENGINE使mysql支持…...
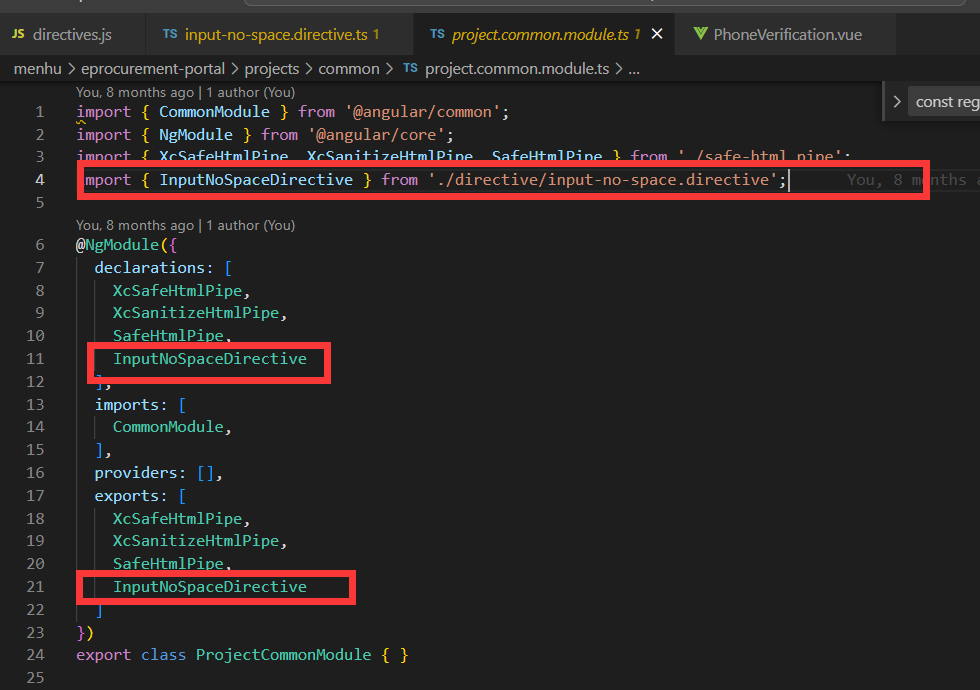
vue自定义指令以及angular自定义指令(以禁止输入空格为例)
哈喽,小伙伴们,大家好啊,最近要实现一个vue自定义指令,就是让input输入框禁止输入空格建立一个directives的指令文件,里面专门用来建立各个指令的官方文档:自定义指令 | Vue.js (vuejs.org)我们都知道vue中…...

异常 复习
异常复习 异常(广义):泛指程序中一切不正常的情况 错误:例如内存不够用,程序是无法解决的 异常(狭义):程序在运行中出现问题,但是可以通过异常处理机制处理,程序可以继续向后执行 异常体系 Throwable类有两个直接子类:Excepti…...
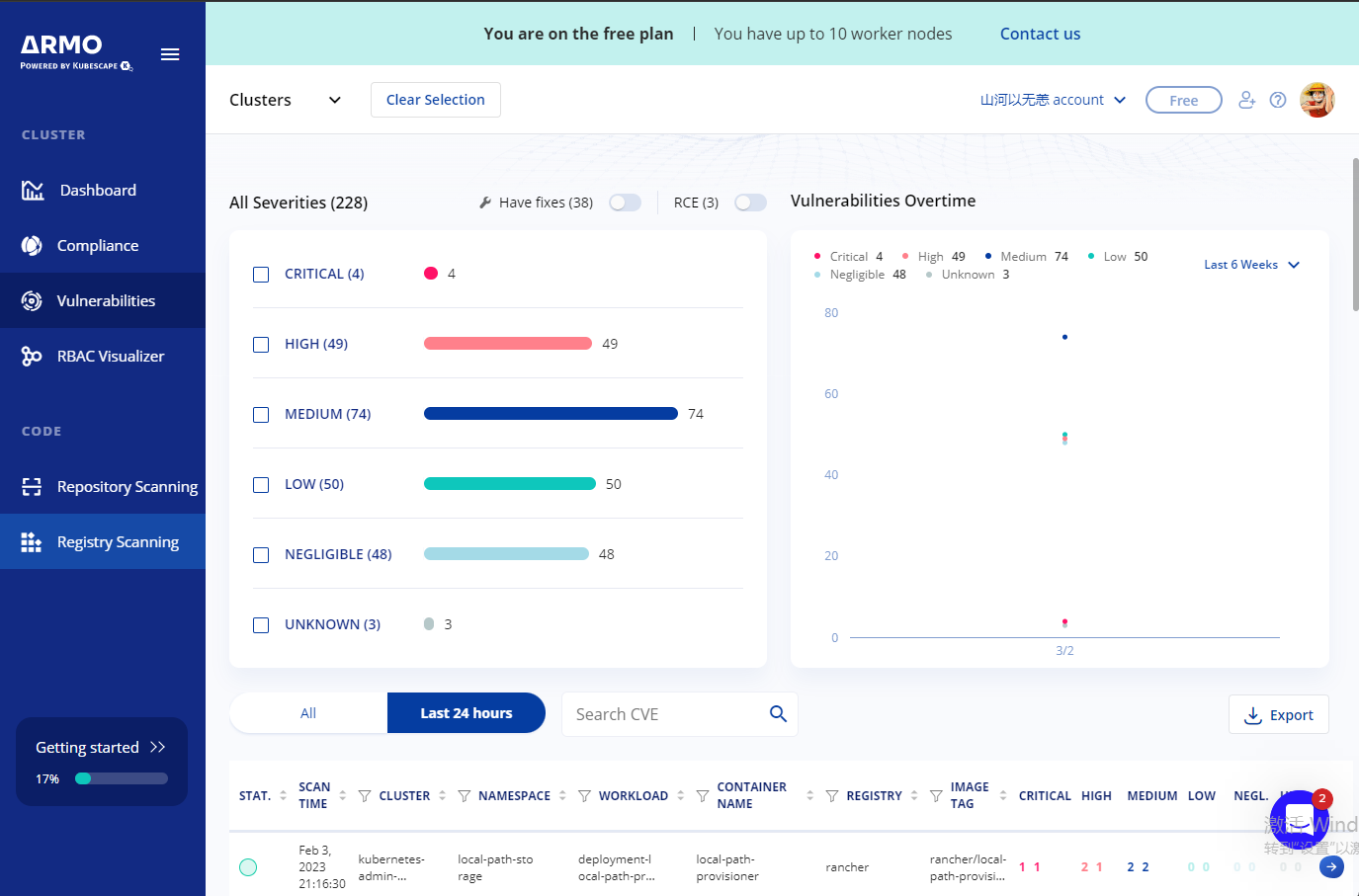
K8s:开源安全平台 kubescape 实现 Pod 的安全合规检查/镜像漏洞扫描
写在前面 生产环境中的 k8s 集群安全不可忽略,即使是内网环境容器化的应用部署虽然本质上没有变化,始终是机器上的一个进程但是提高了安全问题的处理的复杂性分享一个开源的 k8s 集群安全合规检查/漏洞扫描 工具 kubescape博文内容涉及: kube…...

C#中,FTP同步或异步读取大量文件
一次快速读取上万个文件中的内容 在C#中,可以使用FTP客户端类(如FtpWebRequest)来连接FTP服务器并进行文件操作。一次快速读取上万个文件中的内容,可以采用多线程的方式并发读取文件。 以下是一个示例代码,用于读取FT…...
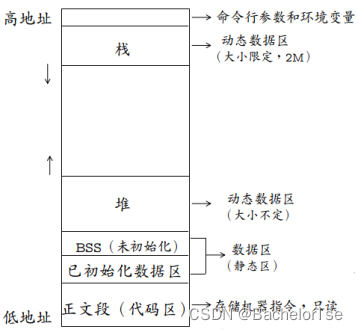
STM32单片机的FLASH和RAM
STM32内置有Flash和RAM(而RAM分为SRAM和DRAM,STM32内为SRAM),硬件上他们是不同的设备存储器、属于两个器件,但这两个存储器的寄存器输入输出端口被组织在同一个虚拟线性地址空间内。 MDK预处理、编译、汇编、链接后编…...

Java 二叉树的遍历
二叉树的遍历(traversing binary tree)是指从根结点出发,按照某种次序依次访问二叉树中所有的结点,使得每个结点被访问依次且仅被访问一次。前序遍历(根 左 右)先访问根结点,然后前序遍历左子树…...

实习日记-C#
数据类型 字符串常量 string a "hello, world"; // hello, world string b "hello, world"; // hello, world string c "hello \t world"; // hello world string d "hello \t wor…...
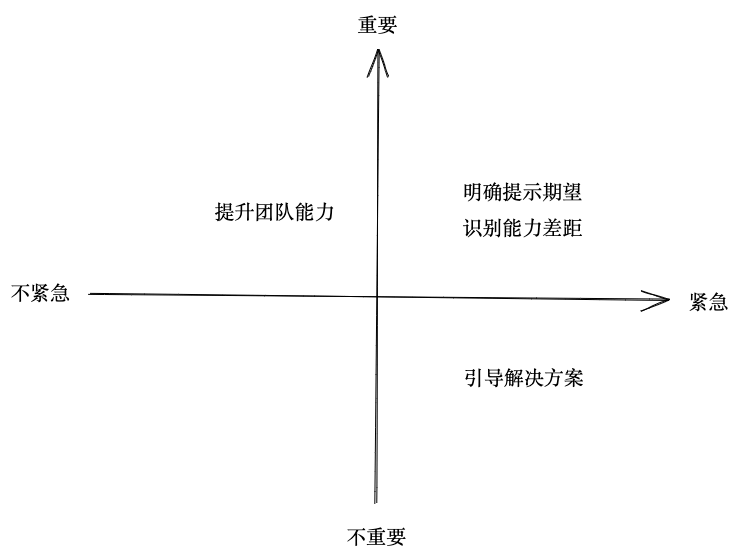
Tech Lead如何引导团队成员解决问题?
作为一个开发团队的Tech Lead,当团队成员向你寻求帮助时,你有没有说过下面这些话? 你别管了,我来解决这个问题你只要。。。就行了你先做其他的吧,我研究一下,然后告诉你怎么做 当我们说这些话时ÿ…...
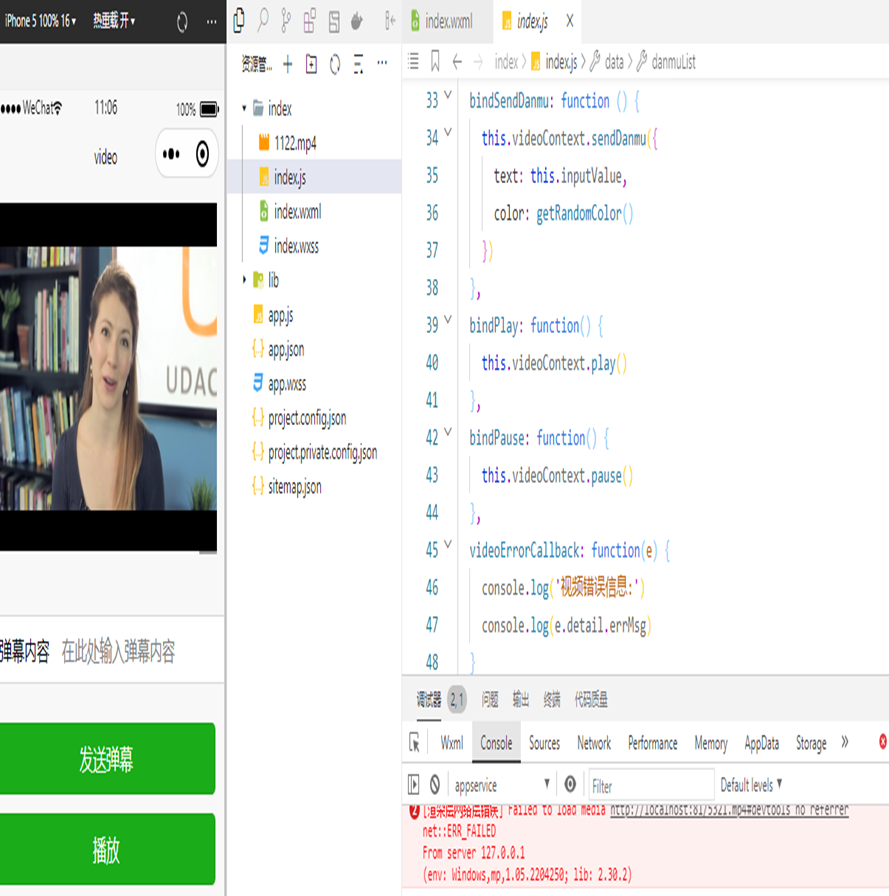
07--组件
一、小程序组件分类微信团队为开发者提供了一系列基础组件,开发者可以通过组合这些基础组件进行快速开发。小程序中的组件也是非常丰富的,开发者可以基于组件快速搭建出漂亮的页面结构。小程序中的组件其实相当于网页中的HTML标签,只不过标签…...

怎么做好一个完整的项目复盘
复盘,是运营必不可少的能力,小到一次买菜的经历,大到百亿千亿的投资项目,都可以通过复盘来总结规律、提升水平。简单说来,复盘可以达到的效果有两条:优化弱项,强化强项明确自己的价值࿰…...